connect的問題,我們搜遍了碩博士論文和台灣出版的書籍,推薦Bright, J. E. (ILT)/ Clever Publishing (COR)寫的 Amazing Animals Activities & Stickers 和Jones, Robin的 History of the Great Central Railway都 可以從中找到所需的評價。
這兩本書分別來自 和所出版 。
國立陽明交通大學 資訊科學與工程研究所 謝秉均所指導 謝秉瑾的 貝氏最佳化的小樣本採集函數學習 (2021),提出 connect關鍵因素是什麼,來自於貝氏最佳化、強化學習、少樣本學習、機器學習、超參數最佳化。
而第二篇論文國立陽明交通大學 電控工程研究所 胡竹生所指導 賴振豪的 基於工件運動配置與環境限制之機器人冗餘度設計與路徑優化抽樣演算法 (2021),提出因為有 冗餘機器人、移動與路徑規劃、取樣方法、優化控制的重點而找出了 connect的解答。
Amazing Animals Activities & Stickers

為了解決 connect 的問題,作者Bright, J. E. (ILT)/ Clever Publishing (COR) 這樣論述:
The colorful pages of AMAZING ANIMALS ACTIVITIES & STICKERS are packed with fluffy mammals, scaly reptiles, swimming sea creatures, and flying birds The entertaining activities include matching games, mazes, word searches, number challenges, decoder games, and fun facts - all designed to keep busy
young minds engaged.With lots and lots of fun-filled stickers, kids will develop hand-eye coordination and early skills as they play.The handy take-along format is perfect for kids on the go J.E. Bright is the writer of more than 120 novels, novelizations, non-fiction books, interactive storybook
apps, and novelty books for children and young adults. He was a children’s book editor for almost 20 years, at publishing companies including Scholastic, HarperCollins, and Simon & Schuster. Now he writes full time.Clever Publishing was founded in 2010 with the purpose of changing children’s lives
for the better. We create a world full of fascinating experiences for families through our books, games, sets, and series. Focusing on Pre-school and Edutainment, we’ve developed a wide range of innovative formats with modern teaching techniques. Kids love to read, touch, and play while learning, so
our program includes products for all ages, including box sets; board books; puzzle books; learning flash cards; interactive coloring, activity, and word play formats; and boards games for the entire family. Our dream team - more than 100 employees worldwide - have a comprehensive understanding and
knowledge of children’s books. With this expertise, we present products that are fun, entertaining, and vibrant. We are modern and educational and strive to always emphasize the importance of first experiences. We connect to the needs of busy parents and aim to enrich the time spent with their chil
dren. Our goal is to make children - as well as their parents happy!
connect進入發燒排行的影片
貝氏最佳化的小樣本採集函數學習
為了解決 connect 的問題,作者謝秉瑾 這樣論述:
貝氏最佳化 (Bayesian optimization, BO) 通常依賴於手工製作的採集函數 (acqui- sition function, AF) 來決定採集樣本點順序。然而已經廣泛觀察到,在不同類型的黑 盒函數 (black-box function) 下,在後悔 (regret) 方面表現最好的採集函數可能會有很 大差異。 設計一種能夠在各種黑盒函數中獲得最佳性能的採集函數仍然是一個挑戰。 本文目標在通過強化學習與少樣本學習來製作採集函數(few-shot acquisition function, FSAF)來應對這一挑戰。 具體來說,我們首先將採集函數的概念與 Q 函數 (Q
-function) 聯繫起來,並將深度 Q 網路 (DQN) 視為採集函數。 雖然將 DQN 和現有的小樣本 學習方法相結合是一個自然的想法,但我們發現這種直接組合由於嚴重的過度擬合(overfitting) 而表現不佳,這在 BO 中尤其重要,因為我們需要一個通用的採樣策略。 為了解決這個問題,我們提出了一個 DQN 的貝氏變體,它具有以下三個特徵: (i) 它 基於 Kullback-Leibler 正則化 (Kullback-Leibler regularization) 框架學習 Q 網絡的分佈(distribution) 作為採集函數這本質上提供了 BO 採樣所需的不確定性並減輕了
過度擬 合。 (ii) 對於貝氏 DQN 的先驗 (prior),我們使用由現有被廣泛使用的採集函數誘導 學習的演示策略 (demonstration policy),以獲得更好的訓練穩定性。 (iii) 在元 (meta) 級別,我們利用貝氏模型不可知元學習 (Bayesian model-agnostic meta-learning) 的元 損失 (meta loss) 作為 FSAF 的損失函數 (loss function)。 此外,通過適當設計 Q 網 路,FSAF 是通用的,因為它與輸入域的維度 (input dimension) 和基數 (cardinality) 無 關。通過廣
泛的實驗,我們驗證 FSAF 在各種合成和現實世界的測試函數上實現了與 最先進的基準相當或更好的表現。
History of the Great Central Railway
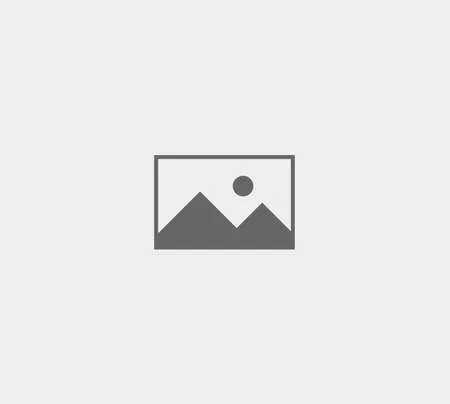
為了解決 connect 的問題,作者Jones, Robin 這樣論述:
The Great Central Railway is the only double track preserved steam railway in the world and its main focus is on the hugely popular modern day line running between Loughborough and Leicester North. Author Robin Jones, editor of Heritage Railway magazine, uses an incredible collection of images fr
om Britain’s top railway photographers to showcase the very best of preserved steam running in an authentic setting. History has its place too with the formation of the original Great Central Railway, built in 1899 to connect the cities of Manchester, Sheffield and London, being explained and examin
ed. Preservation sites set up elsewhere on the former Great Central line are also detailed including those at Buckinghamshire Railway Centre, Elsecar Heritage Railway and Dinting Railway Centre in Glossop
基於工件運動配置與環境限制之機器人冗餘度設計與路徑優化抽樣演算法
為了解決 connect 的問題,作者賴振豪 這樣論述:
由於機械手臂在運動上的多功能性與成本效益,越來越多的加工任務利用它們來執行。為了滿足工件或工具的運動軌跡需求,並且保證加工品質,機器人運動軌跡的複雜性可能太高而無法產生可行的路徑規劃。主要的原因通常是機器人的自由度不足,無法避免奇異點或環境約束。直觀地向機器人添加更多關節並非一個好的解法,因此如何增加機器人冗餘自由度成為一個實際問題。換句話說,添加機器人的冗餘自由度必須考慮任務的運動配置、工具和環境約束。在本文中,提出了一種同時解決機器人的冗餘自由度設計和路徑規劃優化的新方法。機器人冗餘自由度分為兩種類型:設計自由度(design DOF)和運動自由度(motion DOF)。前者代表加工系
統的配置,例如夾具設計和工具/工件放置。後者代表整體運動規劃的參數,包括機器人和周邊設備。針對機器人冗餘自由度的設計和優化路徑規劃的問題,本文以設計自由度與運動自由度開發了不同約束條件下的遞迴優化架構。這個架構在幾何空間下使用取樣的方法在有限時間內搜索優化的解決方案。它的效果透過兩種的案例驗證,機器人研磨和機器人摺邊加工。這兩種案例的模擬結果分別降低了21.73% 和 58.81% 的成本。而且,後者在實驗中降低了55.434%的成本。這個實驗的結果透過視覺感測以平均3.26毫米的精度誤差加以驗證。