Farthest的問題,我們搜遍了碩博士論文和台灣出版的書籍,推薦Chikiamco, Paolo寫的 Muros: Manila Behind Walls: The Case of the Cemetery Girl - A Graphic Novel by Paolo Chikiamco & Borg Sinaban 和Cider Mill Press (COR)的 Discovering Moons Handbook都 可以從中找到所需的評價。
另外網站farther, further 与farthest, furthest 的用法区别与辨析 - 英语语法网也說明:表示具体的距离时,四个词都可用(可用作形容词和副词),但要根据句意确定是用比较级还是最高级。如:. Who walked the farthest [furthest]? 谁走得最远 ...
這兩本書分別來自 和所出版 。
淡江大學 水資源及環境工程學系碩士班 蔡孝忠、蘇仕峯所指導 周立翔的 數值模擬花蓮港之颱風波浪 (2021),提出Farthest關鍵因素是什麼,來自於花蓮港、亞重力波、港池共振、FUNWAVE-TVD。
而第二篇論文國立中正大學 電機工程研究所 賴文能所指導 洪金利的 基於單影像之六自由度物體姿態估測 (2021),提出因為有 的重點而找出了 Farthest的解答。
最後網站'The Farthest': Dublin Review - Screen Daily則補充:Emer Reynolds tracks the Voyager interstellar mission in a cathartic and moving documentary. The Farthest. Dir/scr. Emer Reynolds.
Muros: Manila Behind Walls: The Case of the Cemetery Girl - A Graphic Novel by Paolo Chikiamco & Borg Sinaban
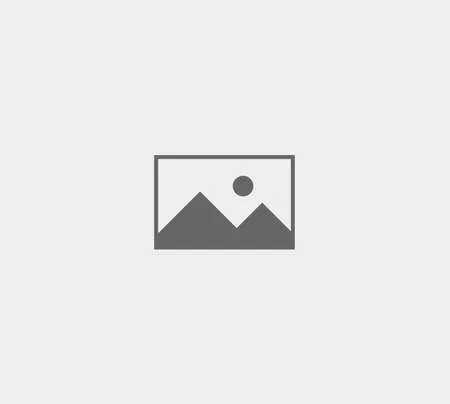
為了解決Farthest 的問題,作者Chikiamco, Paolo 這樣論述:
Paolo Chikiamco was trained as a lawyer but changed paths to establish Eight Ray Sun Publishing in 2009. His articles have appeared in the Philippine Daily Inquirer, Philippine Online Chronicles, and Code RED magazine. Chikiamco has also published stories in The Digest of Philippine Genre Stories,
A Time for Dragons, and The Farthest Shore. He is the co-author of Muros: The Cemetery Girl.Borg Sinaban is an artist, illustrator and graphic designer based in Manil
Farthest進入發燒排行的影片
We are sailing far away to complete a special mission! Once a flourishing fishing village with over 2000 inhabitants, nowadays Grass Island is a sleepy village and a camping spot. This is the farthest I have been since the pandemic. Full of vibrant spring colors and cows, I hope this video brings you joy, laughter and a lot of natural beauty.
遠航來到風光明媚的塔門,除了食海鮮和行山探望牛牛外,我們亦要在一個下午的時間內完成任務!究竟牛牛看到我們執行任務時有乜反應,看看就知道了?
Time: Mid April 2021
Location: Grass Island, Hong Kong
100% shot and created by me with love ❤️
IG: candy_kamanyuen
FB: Candy Kaman Yuen
Email: [email protected]
數值模擬花蓮港之颱風波浪
為了解決Farthest 的問題,作者周立翔 這樣論述:
花蓮港地理位置面臨太平洋容易直接受到颱風波浪影響,颱風期間所引發的長週期亞重力波導致港內港池水位振盪劇烈,港域靜穩度不佳影響船舶作業及停靠安全。港內波高會隨外海波浪之波高、週期及波浪方向不同而改變,為了瞭解不同波浪入射方向之颱風波浪在港內的示性波高及亞重力波,本研究利用FUNWAVE-TVD波浪數值模式模擬2005年龍王颱風之波浪,採用波高7.81公尺及尖峰週期14.2秒之JONSWAP波譜,並分別以ENE向、E向、ESE向及SE向波浪入射花蓮港及其南側海岸,模式與現場觀測比對,加以探討港內平面空間之波浪分佈特性。結果顯示模式能模擬出港內外的亞重力波能量,示性波高僅在外港區較高,內港區相對穩
靜,然而亞重力波在內港區及連結內外港之航道都十分顯著,最大的亞重力波出現在距離港口最遠的內港碼頭。比較 四個波浪方向,ENE向及E向示性波高在外港區相對穩靜,ESE向及SE向入射角度偏南,波浪容易直接入射至港內,示性波高在外港區較高,在內港區四個波浪方向差異性小,但亞重力波的空間分佈差異性大,呈現不同的共振結構,入射波浪ESE向亞重力波能量在港內高於其他波浪方向,入射波浪E向亞重力波能量最低,值得注意的是ENE向與SE向亞重力波能量接近甚至稍高於SE向,表示港內亞重力波能量大小不會因波浪直接入射至港內就越大。本研究之數值模式提供長週期波在港內所有位置之空間分佈,可作為港灣防颱策略與港灣規劃之參
考。
Discovering Moons Handbook
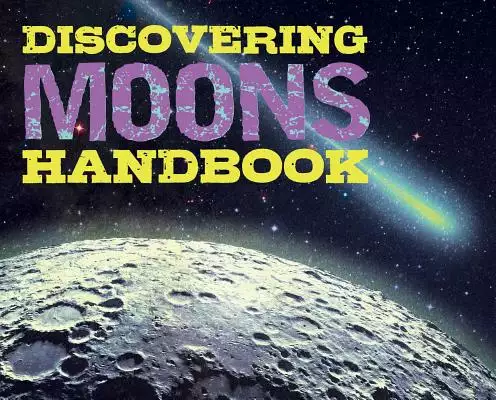
為了解決Farthest 的問題,作者Cider Mill Press (COR) 這樣論述:
Explore the farthest reaches of the universe from the comfort of your living room with the Discovering Moons Handbook. Ever wondered how many moons Jupiter has? Want to know how big our moon is? Then this out-of-this-world handbook will have you over the moon Featuring a tactile, glow-in-the-dar
k cover, and amazing, scientifically-accurate illustrations, this book is the perfect gift for the little astronomer in your life. With facts about moons from Callisto to Charon and everything between, explore the farthest reaches of the universe from the comfort of your living room with the Discove
ring Moons Handbook.
基於單影像之六自由度物體姿態估測
為了解決Farthest 的問題,作者洪金利 這樣論述:
Dealing with the object pose estimation from a single RGB image is very challenging since 6 degree-of-freedom (6DoF) parameters have to be predicted without using the spatial depth information. Since direct regression of the pose parameters by using the deep neural network was reportedly poor and t
hen attaching with the refinement module to improve the accuracy causes much time consumption, in this work, we propose several techniques of top-down or bottom-up approaches to predict indirect feature maps instead from which single or multiple object poses can be recovered by using sophisticated p
ost-processing algorithms.Since there are four possible scenarios where single/multiple objects in the same/different classes can appear in the image, the corresponding output feature maps are predicted differently. For a single object scenario, unit-vector fields are predicted. These features are c
omposed of many unit-vectors pointing from pixels within the object mask to the pre-defined 2D object keypoints where their corresponding 3D object keypoints are distributed optimally on the 3D object surface based on the keypoint distances and object surface curvatures. From some pairs of the predi
cted unit-vectors, 2D projected keypoints can be voted and determined, so that PnP algorithm can be applied to estimate the pose. To deal with multiple objects even in the same or different classes, sufficient and informative output feature maps need to be predicted. Different from object keypoints,
6D coordinate maps which form the main features can be considered as a bunch of 3D point clouds for pose parameter calculation when their 2D-3D correspondences are also established. 6D coordinate maps contains two parts: front- and rear-view 3D coordinate maps. 3D coordinate map is actually a 2D ma
p where each pixel records 3D coordinates of a point in the object CAD model which projects to that 2D pixel location. Via 3D/6D coordinate maps, instance 2D-3D correspondences of a large point set can be built and PnP algorithm combined with RANSAC scheme to overcome the outliers or noise can be us
ed to estimate multiple object poses. Even though in this case, 2D object keypoints can no longer be used to estimate multiple poses, they can be defined as single/multiple reference points for identifying all object instance masks even in the presence of heavy occlusion. We are also interested in o
vercoming some problems related to the missing information and symmetry ambiguity encountered when generating the ground truth of 6D coordinate maps.Our studies show that our single pose estimation method using unit-vector fields can achieve an outstanding accuracy if compared to other top-down stat
e-of-the-art methods without including refinement modules. It has a good algorithm to identify the designated object keypoints from which the predicted feature maps are trained with the effective loss functions, but it has a slower inference speed when multiple object poses are taken into considerat
ion. On the other hand, our 6D coordinate maps, combining with the information from two opposite views, are capable of providing more constraints for network optimization and hence helpful for pose estimation accuracy. Our methods using 6D coordinate maps can achieve great performances if compared t
o other multiple object pose estimation methods.
想知道Farthest更多一定要看下面主題
Farthest的網路口碑排行榜
-
#1.Farthest meaning in Hindi - फरथेस्ट मतलब हिंदी में - Hinkhoj
Farthest meaning in Hindi : Get meaning and translation of Farthest in Hindi language with grammar,antonyms,synonyms and sentence usages. 於 dict.hinkhoj.com -
#2.FARTHEST (adverb) definition and synonyms - Macmillan ...
Definition of FARTHEST (adverb): in or to most distant place; to or at most distant time. 於 www.macmillandictionary.com -
#3.farther, further 与farthest, furthest 的用法区别与辨析 - 英语语法网
表示具体的距离时,四个词都可用(可用作形容词和副词),但要根据句意确定是用比较级还是最高级。如:. Who walked the farthest [furthest]? 谁走得最远 ... 於 www.yygrammar.com -
#4.'The Farthest': Dublin Review - Screen Daily
Emer Reynolds tracks the Voyager interstellar mission in a cathartic and moving documentary. The Farthest. Dir/scr. Emer Reynolds. 於 www.screendaily.com -
#5.farthest中文- 英漢詞典 - 漢語網
farthest 中文的意思、翻譯及用法:adv. 最遠地;最廣地;最先進地adj. 最遠的;最廣闊的。英漢詞典提供【farthest】的詳盡中文翻譯、用法、例句等. 於 www.chinesewords.org -
#6.farthest在線翻譯- 用法_例句 - 海词词典
海詞詞典,最權威的學習詞典,為您提供farthest的在線翻譯,farthest是什麼意思,farthest的真人發音,權威用法和精選例句等。 於 dict.cn -
#7.What does farthest mean? - Definitions.net
Definition of farthest in the Definitions.net dictionary. Meaning of farthest. What does farthest mean? Information and translations of farthest in the most ... 於 www.definitions.net -
#8.farthest - Dictionary Definition : Vocabulary.com
farthest Add to list Share · adjective. (comparatives of `far') most remote in space or time or order · adverb. to the greatest distance in space or time (` ... 於 www.vocabulary.com -
#9.i was farthest from - 英中– Linguee词典
大量翻译例句关于"i was farthest from" – 英中词典以及8百万条中文译文例句搜索。 於 cn.linguee.com -
#10.farthest แปลว่าอะไร ดูความหมาย ตัวอย่างประโยค หมายความว่า ...
[ADJ] ไกลมากที่สุด (ใช้กับเวลา พื้นที่และระยะทาง), See also: ห่างมากที่สุด, Syn. farthermost, furthest · farthest, [ADV] ไกลมากที่สุด (ใช้กับเวลา พื้นที่และระยะ ... 於 dict.longdo.com -
#11.Farthest South & Other Stories: Rutherford, Ethan - Amazon.com
The stories in Farthest South, the second story collection from renowned writer Ethan Rutherford, find characters in the most unexpectedly menacing of ... 於 www.amazon.com -
#12.Dreye權威釋義|farthest
farthest. KK:[ˈfɑrðɪst] DJ:[ˈfɑːðist]. 權威釋義. 英語. a. (距離、時間上)最遠的. the farthest corner. 最邊遠的地方. ad. (距離、時間上)最遠地. 於 yun.dreye.com -
#13.The Farthest Shore | Earthsea Wiki
The Farthest Shore is the third book of the Earthsea series. A strange, inexplicable malaise is spreading throughout Earthsea. Magic is losing its power; ... 於 earthsea.fandom.com -
#14.Scientists Think This May Be the Farthest Galaxy in the Universe
Astronomers have peered out into the vast expanse and spotted what they think is the farthest (and oldest) galaxy ever observed. 於 www.scientificamerican.com -
#15.farthest - 翰林雲端學院
翻譯: 從你學校到警察局有多遠? 變化: 原形: far. 比較級: farther,further. 最高級: farthest,furthest. 片語: ... 於 www.ehanlin.com.tw -
#16.Sort points from closest to farthest - Stack Overflow
That sounds like a minimum spanning tree, try using Prim's algorithm and storing the order of addition in a list you would get that sorting. 於 stackoverflow.com -
#17.What's the farthest you've ever been from home?
Read 30 answers by scientists to the question asked by Aamal Ghazi Mahdi Al-Saadi on Dec 2, 2017. 於 www.researchgate.net -
#18.In the farthest reaches of the earth - Whitestone Gallery
2021Panel, Oil, Acrylic, Alkyd resin, Co-seal19.0 × 27.3cm. 於 www.whitestone-gallery.com -
#19.The Farthest Operating Spacecraft, Voyagers 1 and 2, Still ...
In the NewsThis year marks the 40th anniversary of the launch of the world's farthest and longest-lived spacecraft, NASA's Voyager 1 and 2. 於 www.jpl.nasa.gov -
#20.Nearest and farthest points in spaces of curvature bounded ...
Issues regarding the farthest point problem are somewhat similar and the readers are referred to [1], [5], [6], [13], [20]. For more developments and ... 於 www.sciencedirect.com -
#21.The Farthest | PBS
The epic story of NASA's Voyager mission to the outer planets and into interstellar space. 於 www.pbs.org -
#22.farthest_百度百科
farthest ,英语单词,主要用作形容词、副词,作形容词时意为“最远的;最广阔的”,作副词时意为“最远地;最广地;最先进地”。 於 baike.baidu.com -
#23.farthest 的情境影片範例|影音字典 - VoiceTube
The dyes were particularly expensive and imported the farthest. 201 18. B2 中高級. 在釜山品嚐韓國海味|在韓國吃蠕動的生章魚(Trying EXOTIC KOREAN SEAFOOD in ... 於 tw.voicetube.com -
#24.similarity in Big Five personality predicts more negative marital ...
Birds of a feather don't always fly farthest: similarity in Big Five personality predicts more negative marital satisfaction trajectories in long-term marriages. 於 pubmed.ncbi.nlm.nih.gov -
#25.聽下「farthest」喺英語入面點讀 - Forvo
to the greatest distance in space or time (`farthest' is used more often than `furthest' in this physical sense); to the greatest degree or extent or most ... 於 yue.forvo.com -
#26.BEYOND THE FARTHEST STAR #1 CVR A RANALDI
PREVIEWSworld | Comic Book, Graphic Novel and Pop-Culture Merchandise News, Previews, Release Dates and More. 於 www.previewsworld.com -
#27.The Farthest (2017) - IMDb
The Farthest: Directed by Emer Reynolds. With Frank Drake, Carolyn Porco, John Casani, Lawrence Krauss. It is one of humankind's greatest achievements. 於 www.imdb.com -
#28.Reaching the Farthest Behind: Maternal Health Innovations at ...
Reaching the Farthest Behind: Maternal Health Innovations at the Facility Level. Yuval Cohen. Share Tweet Pin Mail. UNFPA-Tanzania-Picture. “Innovation happens ... 於 www.newsecuritybeat.org -
#29.The Farthest - WNED PBS Video
The epic story of NASA's Voyager mission to the outer planets and into interstellar space. 於 video.wned.org -
#30.The Farthest Shore - Vertebrate Publishing
The Farthest Shore is the story of Alex's solo trek along the remote Cape Wrath Trail. As he journeyed through a vanishing winter, ... 於 www.v-publishing.co.uk -
#31.Difference Between Farthest and Furthest - Differencebetween ...
“Farthest” is a comparative degree of “far.” It is a more measurable quantity. “Farthest” is used as both an adjective and an adverb. If you are talking about ... 於 www.differencebetween.net -
#32.farthest中文意思- 看影片不用背單字
farthest 的中文意思是什麼呢?2021年最常見的用法,有29影片中用到這個單字,並且可一鍵全部播放,快速聆聽各種外國人(真人),講述這個單字,不再是死死的機器發音。 於 sc.yah101.com -
#33.farthest range - 最大測距 - 國家教育研究院雙語詞彙
farthest range. 暫無建議訊息. Copyright © 2012國家教育研究院版權所有建議最佳瀏覽螢幕解析度1024×768 三峽總院區地址:237201新北市三峽區三樹路2號 ... 於 terms.naer.edu.tw -
#34."farthest" 和"furthest " 的差別在哪裡? | HiNative
farthest 的同義字@eezylamay From the Oxford dictionary: Until recently, farther was preferred in reference to physical distance, ... 於 hinative.com -
#35.farthest 的中文翻譯 | 英漢字典
farthest (a.)最遠的,最久的(ad.)最遠,far的最高級. 於 cdict.net -
#36.Farthest yesterday - FUTURE AFTER A SECOND - StreetVoice
Farthest yesterday. Metal・2017-02-03. To the Light ... 分享:. Farthest yesterday ... The past all burned in the flame, the farthest yesterday 於 streetvoice.com -
#37.Farthest - The Free Dictionary
Most distant or remote, as from a center: extreme, farthermost, furthermost, furthest, outermost, outmost, ultimate, utmost, uttermost. The American Heritage® ... 於 www.thefreedictionary.com -
#38.The Farthest - Rotten Tomatoes
critics consensus. Informative, enthusiastic and accessible, The Farthest will inspire even the most grounded of viewers to look up in wonder once in a ... 於 www.rottentomatoes.com -
#39.Farthest definition and meaning | Collins English Dictionary
Farthest definition: Farthest is a superlative form of → far . | Meaning, pronunciation, translations and examples. 於 www.collinsdictionary.com -
#40.The farthest point strategy for progressive image sampling
A new method of farthest point strategy (FPS) for progressive image acquisition-an acquisition process that enables an approximation of the whole image at ... 於 ieeexplore.ieee.org -
#41.Ghost Island Media 鬼島之音 on Twitter: ""The farthest ...
"The farthest distance in the world is a distant loved one." Reach out to your friends and families to cheer them on! 於 twitter.com -
#42.farthest - Sesli Sözlük
{a} or to the greatest distance, the last: to the greatest degree or extent or most advanced stage (`furthest' is used more often than `farthest' in this ... 於 www.seslisozluk.net -
#43.Difference Between Farthest and Furthest (With Table)
'Farthest' is a superlative adjective or adverb of the word 'Far'. It is utilized in the English language to denote distance that is longer than expected. ' ... 於 askanydifference.com -
#44.FARTHEST GATE LIMITED | LinkedIn
FARTHEST GATE LIMITED | 54 位LinkedIn 關注者。FARTHEST GATE LIMITED is an internet company based out of Euston St, London, United Kingdom. 於 tw.linkedin.com -
#45.The Farthest - Voyager in Space on iTunes - Apple
In 1977, NASA launched twin Voyager spacecraft. An emotional, cinematic documentary, The Farthest tells their story through first-hand accounts ... 於 itunes.apple.com -
#46.'The Farthest' Review - The Hollywood Reporter
Emer Reynolds' documentary 'The Farthest' recounts the continuing story of the Voyager space mission. 於 www.hollywoodreporter.com -
#47.Farthest Field | Raghu Karnad | W. W. Norton & Company
I have not lately read a finer book than this—on any subject at all. . . . A masterpiece.” —Simon Winchester, New Statesman, Farthest Field, An Indian Story ... 於 wwnorton.com -
#48.FARTHEST - Translation in Indonesian - bab.la
Translation for 'farthest' in the free English-Indonesian dictionary and many other Indonesian translations. 於 en.bab.la -
#49.farthestの意味・使い方・読み方 | Weblio英和辞書
(距離・空間・時間が)最も遠く. ... (程度が)極端に 《☆【比較】 この意味では furthest が一般的》. ... [far の最上級] (距離的に)最も遠い. 於 ejje.weblio.jp -
#50.farthest-翻译为中文-例句英语
Industry's fastest and farthest imaging engines deliver superior motion tolerance and barcode read performance. 业内最快最远的影像扫描引擎将为您带来卓越的 ... 於 context.reverso.net -
#51.Beyond Farthest Point Sampling in Point-Wise Analysis - arXiv
Unlike the widely used sampling technique, Farthest Point Sampling (FPS), we propose to learn sampling and downstream applications jointly. 於 arxiv.org -
#52.Etymology, origin and meaning of farthest by etymonline
FARTHEST Meaning: "most distant or remote," late 14c., superlative of far. See definitions of farthest. 於 www.etymonline.com -
#53.Earth farthest from the sun on July 5 | Astronomy Essentials
Earth is farthest from the sun for all of 2021 on July 5. Astronomers call this point in our orbit "aphelion." 於 earthsky.org -
#54.Farther, further or farthest , furthest ? 不再分不清! - ASO ...
說實話,連很多母語人士也會不小心混用farther, further 跟farthest , furthest,不過它們之間還是有正確性上的差異,來看如何使用才不會出錯吧! 於 asoenglishschool.com -
#55.在"英语"词典里farthest}的意思 - Educalingo
在英语词典里带使用范例的farthest含义farthest的近义词以及farthest的25种语言翻译。 於 educalingo.com -
#56.DELUHI - The farthest (英/繁中字幕) 影片Dailymotion
DELUHI - The farthest (英/繁中字幕) 於 www.dailymotion.com -
#57.Farthest Definition & Meaning | Dictionary.com
farthest · at or to the greatest distance. · at or to the most advanced point. · at or to the greatest degree or extent. 於 www.dictionary.com -
#58.The Farthest - 首頁| Facebook
The Farthest 。 4004 個讚。 Official Facebook Page for feature documentary film THE FARTHEST about the iconic Voyager space mission and its Golden Record. 於 zh-tw.facebook.com -
#59.Weather Frobisher's Farthest - meteoblue
Today's and tonight's professional weather forecast for Frobisher's Farthest. Precipitation radar, HD satellite images, and current weather warnings, ... 於 www.meteoblue.com -
#60.When Neptune became the farthest... - The Hindu
While Neptune might now have the right to claim being the planet farthest from the sun in our solar system, it wasn't the case always. 於 www.thehindu.com -
#61.Reaching the Farthest First: The State of Social Enterprise in ...
Reaching the Farthest First: The State of Social Enterprise in the Philippines. The number of social enterprise start-ups has tripled in the last decade. 於 www.britishcouncil.ph -
#62.The Farthest 歌詞Deluhi ※ Mojim.com - 魔鏡歌詞
The Farthest I was chasing my hope. Deep inside me. Deep inside me. But it can't be found! Grief inside me! Grief inside me! I quake, I break. 於 mojim.com -
#63.Farthest Meaning | Best 13 Definitions of Farthest
The definition of farthest is something that is a longer, measurable physical distance. An example of farthest is Pluto, the farthest known body from the sun in ... 於 www.yourdictionary.com -
#64.farthest中文(繁體)翻譯:劍橋詞典
The farthest landmark visible is about 30 miles away. 能看見的最遠的地標大約有30公里遠。 (farthest在劍橋英語-中文(繁體)詞典的翻譯© Cambridge University ... 於 dictionary.cambridge.org -
#65.farthest和furthest的区别用法是什么 - 星火网校
farthest. furthest. 同学们在英语单词的学习过程中经常会遇到多个单词,它们都表示相同或相似的含义,这些单词属于同近义词,在含义上相似但是在用法上存在区别,今天 ... 於 www.xhwx100.com -
#66.Farthest Frontier on Steam
Farthest Frontier. Developer. Crate Entertainment. Publisher. Crate Entertainment. Released. 2022. Protect and guide your people as you forge a town ... 於 store.steampowered.com -
#67.farthest中文, farthest是什麼意思:最遠… - 查查在線詞典
farthest 中文::最遠…,點擊查查權威綫上辭典詳細解釋farthest的中文翻譯,farthest的發音,音標,用法和例句等。 於 tw.ichacha.net -
#68.farthest 中文意思是什麼 - TerryL
farthest 解釋. adj. 形容詞 最遠的,頂遠的。 the farthest corner of the country 最遙遠的角落;最偏遠的地方。 adv. 動詞 副詞 最遠;最大程度地,最大限度地。 於 terryl.in -
#69.The Farthest | RMPBS
The epic story of NASA's Voyager mission to the outer planets and into interstellar space. 於 video.rmpbs.org -
#70.The Farthest Edge - National Parks Conservation Association
The Farthest Edge ... Chasing solitude — and Thoreau — on the Outer Beach of Cape Cod National Seashore. At the crest of the first dune, the pickup halted, then ... 於 www.npca.org -
#71.farthest - Longman Dictionary
farthest · farthestfar‧thest1 /ˈfɑːðɪst $ ˈfɑːr-/ adverb · FARat or to the greatest distance away; a superlative form of 'far' SYN furthest My sister was the one ... 於 www.ldoceonline.com -
#72.What Is the Farthest Object in the Solar System? | VPM
Let's go far far away. To the farthest observed thing in our solar system, a whopping 132 times further from the sun than you are right now. 於 vpm.org -
#73.farthest的中文意思翻譯和英語場景例句- 留聲詞典 - 留声词典
farthest 的中文意思翻譯和英語場景例句。 ... farthest. farthest. * 未能查詢到該單詞. 回到顶部 © 2021 留聲英語., All Rights Reserved. 關於我們聯繫我們隱私政策. 於 dictionary.liushengyingyu.com -
#74.farthest - Wiktionary
Hyphenation: far‧thest. AdjectiveEdit. farthest. Alternative form of furthest. (See also usage notes at further.) AdverbEdit. farthest. 於 en.wiktionary.org -
#75.Find the farthest smaller number in the right side
arr[4] is the farthest smallest element to the right of arr[2]. And for the rest of the elements, there is no smaller element to their right. 於 www.geeksforgeeks.org -
#76.farthest | English Definition & Examples - Ludwig Guru
Companies in Europe have traditionally begun rationalisation farthest from home, by lopping off their branch factories and repatriating production to save ... 於 ludwig.guru -
#77.Farthest - Definition for English-Language Learners from ...
Definition of farthest written for English Language Learners from the Merriam-Webster Learner's Dictionary with audio pronunciations, usage examples, ... 於 www.learnersdictionary.com -
#78.Farthest or Furthest: Which is Correct? - School & Travel
At times words like "Farthest or Furthest" can be a bit confusing as they can seem to have the same meaning. In this context, they both. 於 schoolandtravel.com -
#79.Farthest Definition & Meaning - Merriam-Webster
Definition of farthest · 1 : to or at the greatest distance in space or time who can jump the farthest · 2 : to the most advanced point goes farthest toward ... 於 www.merriam-webster.com -
#80.Farthest South & Other Stories a book by Ethan Rutherford
Foxes raise and then lose a human child. A man, in the final throes of his deathbed fever-dream, experiences a cross-Antarctic voyage. The stories in Farthest ... 於 bookshop.org -
#81.The Farthest - Instrumental-Graupel-KKBOX
Graupel的歌曲「The Farthest - Instrumental」在這裡,快打開KKBOX 盡情收聽。 於 www.kkbox.com -
#82.The Farthest Known Supernova: Support for an Accelerating ...
The Farthest Known Supernova: Support for an Accelerating Universe and a Glimpse of the Epoch of Deceleration *. Adam G. Riess 1, Peter E. Nugent 2, ... 於 iopscience.iop.org -
#83.Aphelion Day: The Earth will be at its farthest point from the Sun
The Earth will be at its farthest point from the Sun in its orbit at 5:27 PM today. 於 www.knoe.com -
#84.farthest - WordReference.com 英汉词典
farthest - WordReference.com 英汉词典. ... 本页中: farthest, far, furthest ... On a clear day, you can see the farthest mountain from here. 於 www.wordreference.com -
#85.farthest - Yahoo奇摩字典搜尋結果
far · adj. 遠的;遙遠的;久遠的;較遠的;那一邊的;對面的[B][Z] · adv. 遠;遙遠地;久遠地;到很深的程度;到很遠的距離[(+into)] · n. 遠方;遠處[U] ... 於 tw.dictionary.yahoo.com -
#86.Review: A True-Life Journey Into Interstellar Space in 'The ...
The Farthest: NYT Critic's Pick: Directed by Emer Reynolds: Documentary, History: PG: 2h 1m. By Andy Webster. Aug. 10, 2017. 於 www.nytimes.com -
#87.farthest - Oxford Learner's Dictionaries
Definition of farthest adverb from the Oxford Advanced Learner's Dictionary ... a competition to see who could throw (the) farthest; farthest (away) (from ... 於 www.oxfordlearnersdictionaries.com -
#88.The Farthest Shore (Earthsea Cycle, #3) by Ursula K. Le Guin
Together they will sail to the farthest reaches of their world -- even beyond the realm of death -- as they seek to restore magic to a land desperately ... 於 www.goodreads.com -
#89.farthest - Meaning in Hindi - Shabdkosh
farthest - Meaning in Hindi, what is meaning of farthest in Hindi dictionary, pronunciation, synonyms and definitions of farthest in Hindi and English. 於 www.shabdkosh.com -
#90.The Farthest - Wikipedia
The Farthest is an Irish documentary film that chronicles the history of the Voyager program and its two space probes, Voyager 1 and Voyager 2, ... 於 en.wikipedia.org -
#91.The farthest galaxy in the universe | The University of Tokyo
The farthest galaxy in the universe Chemical signatures give away the distance to the most distant galaxy Research news. 於 www.u-tokyo.ac.jp -
#92.Farthest South & Other Stories - Deep Vellum Publishing
By Ethan Rutherford Rising literary star Ethan Rutherford's fevered new collection pulls together eight short stories about family and home that are at the ... 於 store.deepvellum.org -
#93.farther、 further、 farthest和furthest用法辨析 - 每日頭條
1.To talk about distance, use either farther, farthest or further, furthest . In BrE , further , furthest are the most common forms and in ... 於 kknews.cc -
#94.Farthest North - 博客來
書名:Farthest North,語言:英文,ISBN:9783752434835,頁數:190,作者:Lanman, Charles,出版日期:2020/08/14,類別:文學. 於 www.books.com.tw -
#95.Fairbanks Girl Scouts | Farthest North Girl Scout Counsel
Farthest North Girl Scout Council. COVID-19 and Girl Scouting. – UPDATED september 27, 2021 ... 於 fairbanksgirlscouts.org -
#96.英语-汉语farthest翻译
'farthest'在免费英语-汉语词典的翻译,查看更多汉语的翻译。 於 www.babla.cn