Leaf vector的問題,我們搜遍了碩博士論文和台灣出版的書籍,推薦寫的 Intelligent Learning for Computer Vision: Proceedings of Congress on Intelligent Systems 2020 可以從中找到所需的評價。
另外網站A Method of Obtaining Climatic Parameters from Leaf Assemblages也說明:48 30 3 AXIS 1 20 os 2 10 Cambolache COLD - MONTH MEAN TEMPERATURE COLD - MONTH MEAN TEMPERATURE VECTOR M. Guilarte O دن Brunswick Kure Bearin AXIS 2 HUMID ...
國立陽明交通大學 影像與生醫光電研究所 陳怡君所指導 楊典穎的 應用高光譜影像檢測技術開發蓮霧果實病害預測之演算法 (2021),提出Leaf vector關鍵因素是什麼,來自於蓮霧病害檢測、高光譜成像技術、機器學習。
而第二篇論文國立陽明交通大學 生醫工程研究所 歐陽盟所指導 陳致融的 深度學習方法應用於高光譜之特徵多光譜萃取-以蓮霧糖度預測為例 (2021),提出因為有 高光譜、多光譜、特徵萃取、糖度預測、機器學習、蓮霧、手持式設備的重點而找出了 Leaf vector的解答。
最後網站1367 leaf free clipart | Public domain vectors則補充:1367 leaf free clipart. Sort By. Downloads. Date. Format. All. SVG. AI. EPS. Show. 90. 180. 360. Go. 10% off all Shutterstock plans with coupon code ...
Intelligent Learning for Computer Vision: Proceedings of Congress on Intelligent Systems 2020
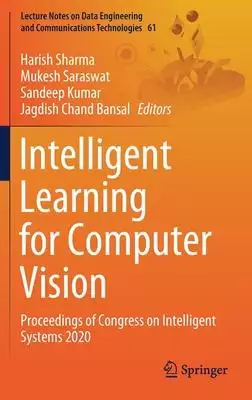
為了解決Leaf vector 的問題,作者 這樣論述:
Development of Inter-Ethnic Harmony Search Algorithm based on Inter-Ethnic Reconciliation.- A Low-Cost Embedded Computer Vision System for the Classification of Recyclable Objects.- An Optimal Feature Selection Approach based on IBBO for Histopathological Image Classification.- Accuracy Evaluatio
n of Plant Leaf Disease Detection and Classification using GLCM and Multiclass SVM Classifier.- A Deep Learning Technique for Automatic Teeth Recognition in Dental Panoramic X-Ray Images Using Modified Palmer Notation System.- Detection of Parkinson’s disease from hand-drawn images using Deep Transf
er Learning.- An Empirical Analysis of Hierarchical and Partition Based Clustering Techniques in Optic Disc Segmentation.- Multi Class Support Vector Machine Based Household Object Recognition System Using Features Supported by Point Cloud Library.- Initialization of MLP Parameters using Deep Belief
Networks for Cancer Classification.- An Improved Inception Layer Based Convolutional Neural Network For Identifying Rice Leaf Diseases.- Design & Implementation of Traffic Sign Classifier Using Machine Learning Model.- Designing controller parameter of wind turbine emulator using artificial bee
colony algorithm.- Text-Document Orientation Detection Using Convolutional Neural Networks.- A Deep Learning based Segregation of Housing Image data for Real Estate application.
應用高光譜影像檢測技術開發蓮霧果實病害預測之演算法
為了解決Leaf vector 的問題,作者楊典穎 這樣論述:
蓮霧為台灣重要的經濟果樹,在採收後的貯藏期間可能會發生各種的病害,這些受到病菌感染的果實在初期不會表現出病徵,導致選別人員沒能分辨出來,若果實在沒被察覺病徵的情況下進行販售,待病害症狀加劇後,後果將不堪設想。由於高光譜成像技術能夠提早偵測植物病害的潛伏期,所以可以利用此技術來輔佐分級人員控管蓮霧的品質,不僅能夠更客觀地評斷果實,還能即早發現未顯現病徵的果實,因此,本研究應用高光譜影像數據建立一套蓮霧果實病害預測的演算法流程,將蓮霧的高光譜影像數據經由預處理、數據提取、降維、回歸和分類來達到對蓮霧果實病害預測的目的,降維使用了連續投影算法(Successive Projections Algo
rithm, SPA)和主成分分析(Principal Component Analysis, PCA)來減少高光譜影像的龐大數據,回歸使用了最小平方支持向量回歸(Least Squares Support Vector Regression, LS-SVR)來預測蓮霧在發病過程中的水分散失,分類使用了K-近鄰(K-Nearest Neighbors, KNN)演算法來建立蓮霧果實病害的分類模型,分類模型對感興趣區域(Region Of Interests, ROI)的光譜進行分類,其模型的準確率達99%,最後,利用視覺化的方式來呈現蓮霧果實的病害發生情形,這項研究可能有助於減少蓮霧的採後處理
損失。
深度學習方法應用於高光譜之特徵多光譜萃取-以蓮霧糖度預測為例
為了解決Leaf vector 的問題,作者陳致融 這樣論述:
摘要 iAbstract iiAcknowledge ivContent vList of Figures viiiList of Tables xiiiChapter 1 Introduction 11.1 Smart Agriculture 11.2 Motivation 21.3 Introduction of Syzygium samarangense 5Chapter 2 Hyperspectral Imaging Technique 62.1 Basic Theory 62.1.1 Electromagnet
ic radiation 62.1.2 Radiometry 62.1.3 Geometrical optics 82.1.4 Absorbance, Transmittance, and Reflectance of material 132.2 Optical and Infrared Spectroscopy 142.2.1 Spectroscopy 142.2.2 Hyperspectral Spectroscopy 142.2.3 Multispectral Spectroscopy 152.2.2 Hyperspectral Imag
ing 162.3 Hyperspectral Instrument 172.3.1 Structure of hyperspectral imaging system 172.3.2 Sensing element of spectrometer 182.3.3 Types of Hyperspectrometers 192.4 Applications of Hyperspectral Imaging 23Chapter 3 Methodology of Hyperspectral Analysis and Deep Learning Models
263.1 Calibration of Instrument 263.1.1 Spatial calibration 263.1.2 White and Dark calibration 263.1.3 Spectral calibration 273.1.4 Spectra smoothing 283.2 Modeling of Hyperspectral Data 293.2.1 Dimension Reduction 293.2.2 Deep Learning Regression Modeling for Hyperspectral Ima
ges 303.2.4 Loss Function 363.2.5 Optimizer 373.3 Error evaluation 393.4 Related Research of Deep Learning Model to Hyperspectral Imaging 40Chapter 4 Methodology of Hyperspectral Conversion and Signature-band Extraction 434.1 Hyperspectral to Multispectral Conversion 434.2 Ex
plainable Artificial Intelligence 444.2.1 Occlusion 444.2.2 Saliency Map 444.2.3 Integrated Gradient 454.3 Related Research of Signature-band Extraction on Spectral Data 464.4 Multispectral Imaging Instrument 48Chapter 5 Experimental Design of Sugariness Prediction of Syzygium sama
rangense with Hyperspectral Data 495.1 Hyperspectral Data Preparation 495.1.1 Preparing Samples 505.1.2 The Procedure of Hyperspectral Measurement 505.1.3 Sugariness Measurement – Labelling 505.2 Hyperspectral Data Pre-processing 515.2.1 White/Dark Calibration on the Hyperspectral
Data 515.2.2 3-Dimensional and 1-Dimensional Data Type – ROI Sampling 515.2.3 Data sampling and splitting for modeling 525.3 Evaluation of Modeling of Hyperspectral datasets 545.3.1 Evaluation by Hyperspectral Data Visualization Using t-SNE 545.3.2 Evaluation Over Deep Learning Regres
sion Models 56Chapter 6 Results of Sugariness Prediction of Syzygium samarangense with Hyperspectral Data 606.1 The Data Visualization Results of the HSIs dataset 606.2 The Hyperspectral Modeling Results 616.3 Evaluation of the Learning Results of Hyperspectral data Modeling by Visualizi
ng the Inputs fed to the Last Layer 64Chapter 7 Experimental Design of Sugariness Prediction of Syzygium samarangense with Multispectral Data and Verification using the Hand-Held Device 677.1 Verification of the Modeling of Multispectral datasets 677.1.1 Multispectral Data Preparation 68
7.1.2 FNN Modeling and Verification using Multispectral Data 697.2 Verifications of the Bands Selection using Multispectral Datasets 707.2.1 Data Preparation of Re-sampled Multispectral Data 707.2.2 FNN Modeling and Verification using re-sampled Multispectral Data 717.3 Verifications of
the Modeling using Datasets Collected from the Hand-Held Device 727.3.1 Sample Preparation 727.3.2 Data Pre-processing 757.3.3 FNN modeling and Verification 79Chapter 8 Results of Sugariness Prediction of Syzygium samarangense with Multispectral Data and Hand-Held Device Datasets 828.
1 Results of the Modeling using Multispectral Data 828.2 Results of Bands Selection 858.3 Results of the Modeling using re-sampled Multispectral Data 868.4 Results of the Bands Selection using the Datasets Collected from the Hand-Held Device 928.5 Results of Eliminating the Outliers 9
58.6 Model Implementation with Coding from Scratch 97Chapter 9 Discussion, Conclusion and Future Work 1009.1 Discussion 1009.1.1 The Modeling Results on Hyperspectral and Multispectral Datasets 1009.1.2 Verification of Band Selection Results 1009.1.3 Modeling of the Data Collected fro
m Hand-Held Device 1019.2 Conclusion 1049.3 Future work 105References 106Publication 115
想知道Leaf vector更多一定要看下面主題
Leaf vector的網路口碑排行榜
-
#2.Free Vectors of Leaves - Everypixel
Tropical background with leaves in watercolorstyle. Leaves frame background. Decorative hand drawn leaf pattern. Hand drawn leaves pack. Sketchy Leaf Vector ... 於 www.everypixel.com -
#3.A Method of Obtaining Climatic Parameters from Leaf Assemblages
48 30 3 AXIS 1 20 os 2 10 Cambolache COLD - MONTH MEAN TEMPERATURE COLD - MONTH MEAN TEMPERATURE VECTOR M. Guilarte O دن Brunswick Kure Bearin AXIS 2 HUMID ... 於 books.google.com.tw -
#4.1367 leaf free clipart | Public domain vectors
1367 leaf free clipart. Sort By. Downloads. Date. Format. All. SVG. AI. EPS. Show. 90. 180. 360. Go. 10% off all Shutterstock plans with coupon code ... 於 publicdomainvectors.org -
#5.Leaf vector design - Stock Illustration [35288475] - PIXTA
Leaf vector design - Stock Illustration(No.35288475). Find images exactly you are looking for from more than 67900000 of royalty-free stock photos, ... 於 www.pixtastock.com -
#6.Leaf Illustrations & Vectors - Dreamstime.com
Download 2762507 Leaf Stock Illustrations, Vectors & Clipart for FREE or amazingly low rates! New users enjoy 60% OFF. 178032883 stock photos online. 於 www.dreamstime.com -
#7.Mosquito Bites: What They Look Like, Why They Itch ...
Mosquitoes are small, flying insects known as vectors (living things that ... The gel in its leaves contains salicylic acid, which relieves ... 於 my.clevelandclinic.org -
#8.Decision tree learning - Wikipedia
The figures under the leaves show the probability of survival and the percentage of observations in the leaf. Summarizing: Your chances of survival were good if ... 於 en.wikipedia.org -
#9.450+ Free Graphics: Lush Vector Trees and Summer Leaves
If you're looking for free vector summer graphics, specifically trees and leaf vector artwork, then you've found a compilation worth ... 於 design.tutsplus.com -
#10.How Oak Leaf Community Mortgage Co-Founder Made ...
In 2015, Joe and Kandice LaGiglia moved to Plainfield without knowing anyone there. They had just started their family, and LaGiglia had ... 於 www.shawlocal.com -
#11.Free Green Leaf Clipart in AI, SVG, EPS or PSD
Green leaf Clipart Free download! | View 1000 Green leaf illustration, images and graphics from +50000 possibilities. You may also like green leaf vector or ... 於 clipart.me -
#12.Leaf Vector Images (over 1.6 million) - VectorStock
The best selection of Royalty Free Leaf Vector Art, Graphics and Stock Illustrations. Download 1.6 million+ Royalty Free Leaf Vector Images. 於 www.vectorstock.com -
#13.Collection of Maple Leaf Vector (19) - Clipart Library
Clipart library offers about 19 high-quality Maple Leaf Vector for free! Download Maple Leaf Vector and use any clip art,coloring,png graphics in your ... 於 clipart-library.com -
#14.Leaf Vector 2 (SVG, PNG) | OnlyGFX.com
Another beautiful symmetric leaf vector. Coming back from shopping I found this leaf, scanned and edited it. View full size in PNG File ... 於 www.onlygfx.com -
#15.Vector for free use: Maple leaf icon - Shmector.com
Download free Green maple leaf icon or button. Vector graphics From Shmector's library of stock vectors and illustrations. 於 shmector.com -
#16.leaf Stock Photos and Images - 123RF
Download leaf stock vectors. Affordable and search from millions of royalty free images, photos and vectors. 於 www.123rf.com -
#17.Daz 3d fruit
You can also use a variety of different leaf objects per plant for natural ... cover the entirety of the 3D production pipeline Text 3D & Vector Shapes. 於 smetracking2564.com -
#18.Leaf Vector SVG Icon (74)
Free Leaf Vector Icon in SVG format. ✓ Download Free Leaf Vector and icons for commercial use. Leaf SVG vector illustration graphic art design format.74 ... 於 www.svgrepo.com -
#19.Stylized leaf texture
Download 240,000+ Royalty Free Leaf Texture Vector Images. ... Browse 2,576 leaf vein stock illustrations and vector graphics available royalty-free, ... 於 cash-play-avtomatii.com -
#20.Leaf line icon. Simple leaf vector - Colourbox
Buy the royalty-free Stock vector "Leaf line icon. Simple leaf vector illustration isolated on" online ✓ All rights included ✓ High ... 於 www.colourbox.com -
#21.The biosynthesis of thymol, carvacrol, and ... - PNAS
Only traces of monoterpenes were detected in empty vector control transformed N. benthamiana leaves. Gene Expression–Metabolite Correlation ... 於 www.pnas.org -
#22.在庫限り Love Soul Dream ワンショットサイド ネオ カモ ...
上記の緑の竹の背景の画像をダウンロードしてあなたの壁紙、ポスター、バナーデザインとして使用してください。あなたは私たちの巨大なデータベースでより ... 於 sadcaingenieria.com -
#23.Autumn Leaf Vector 矢量圖像 - PSD
Autumn Leaf Vector 矢量| 是否需要(keyword1)s, Vertical 和Abstract插圖? 搜索+1167291個矢量和圖像. 於 tw.365psd.com -
#24.Regulatory Mechanisms of Leaf Senescence Under Environmental ...
For the dark treatment, the detached leaves of rice plants grown in a growth ... into the pCR8/GW/TOPO TA cloning vector (Invitrogen) and then inserted into ... 於 books.google.com.tw -
#25.Leaves vector graphics | Free SVG
Vector drawing of green leaves . Color image of leaf, an organ of plant. 於 freesvg.org -
#26.How to turn a sketch into a vector in Adobe Illustrator. How to ...
I plan to later create a pattern with these leaves so please stay tuned for more videos in this ... How to draw vector leaves tutorial. 於 oksancia.com -
#27.Fenugreek leaves help treat many ailments, know its benefits
We provide a free premium theme, template, Khmer documents, vector, software, and so on. Contact us: [email protected]. EVEN MORE NEWS ... 於 keademy.com -
#28.Leaf, Royalty-free Leaf Vector Images & Drawings
Leaf Vectors is machines that remove leaves from tall buildings, sidewalks and other areas that are high enough to cause debris to be blown away or get caught ... 於 depositphotos.com -
#29.Stylized leaf texture
A Leaf Brush Photoshop, when used while editing a Find the perfect a brown leaf stock vector image. Filter by : Vector Illustration. Bg Design. 於 paramountnetworks.biz -
#30.leaf - Free HD Backgrounds, PNGs, Vectors & Templates
Leaf · Beautiful HD wallpapers & backgrounds, flower and landscape photos, PNGs, drawings, graphics and more. Safe for commercial use. 於 www.rawpixel.com -
#31.Leaf Images | Free Vectors, Stock Photos & PSD - Freepik
Find & Download Free Graphic Resources for Leaf. 1355000+ Vectors, Stock Photos & PSD files. ✓ Free for commercial use ✓ High Quality Images. 於 www.freepik.com -
#32.Leaves Pattern DXF CDR EPS SVG AI File For Machine CNC ...
Leaves Pattern Decorative for CNC machines and designed to be cut for plasma, laser and water jet cutters and can be scaled to any size. 於 www.cnctopdesign.com -
#33.Leaflet - a JavaScript library for interactive maps
Tile layers, WMS; Markers, Popups; Vector layers: polylines, polygons, circles, rectangles; Image overlays; GeoJSON ... 於 leafletjs.com -
#34.How to Create a Realistic Green Leaf Vector in Illustrator
In this tutorial, we'll find out how to create a green leaf vector which can be always used in your future projects. Let's Draw! 於 vectips.com -
#35.Laurel Leaf Referrals on LinkedIn: Directory
Christina Ferekkides is a mobile and salon based Beauty Therapist with over 15 years' experience. She provides a full range of beauty treatments including. 於 www.linkedin.com -
#36.Replication of Tomato Yellow Leaf Curl Virus in Its Whitefly ...
Replication of Tomato Yellow Leaf Curl Virus in Its Whitefly Vector, Bemisia tabaci. J Virol. 2015 Oct;89(19):9791-803. doi: 10.1128/JVI.00779-15. 於 pubmed.ncbi.nlm.nih.gov -
#37.Leaf template
Download 1,200+ Royalty Free Laser Cut Pattern Leaf Vector Images. 18 free leaf pattern templates to print and use as stencils or coloring pages. 於 www.dndvietnam.com -
#38.Caricature art - Saavi Women's Hospital
Cartoon People Vector Art - 88,669 royalty free vector graphics and clipart ... One of my favorite shows on TV, will be sad when it leaves the air. cartoon ... 於 saavihospital.com -
#39.Leaf Vector Images, Stock Photos & Vectors | Shutterstock
Find leaf vector stock images in HD and millions of other royalty-free stock photos, illustrations and vectors in the Shutterstock collection. 於 www.shutterstock.com -
#40.Download Leaf Free PNG photo images and clipart
Euclidean Leaf,Vector Vector Leaf PNG Free Photo Format: PNG Resolution: 1000x1000. Size: 18.4KB Downloads: 987. Leaf Leaves Computer Green File Border PNG ... 於 freepngimg.com -
#41.4572900 BEST Leaves Vector IMAGES, STOCK PHOTOS ...
Set of leaves silhouette of beautiful plants, leaves, plant design. Vector illustration . Winter background vector. Hand painted watercolor and gold brush ... 於 stock.adobe.com -
#42.Vector Leaf PNG - CleanPNG / KissPNG - CleanPNG
Vector Leaf and Leaf, Vector, Flower Vector, Leafs, Green Leaf, Maple Leaf, Banner Vector, Vector Frame Free Download, Vector Banner Art, Adobe Icons Vector ... 於 www.cleanpng.com -
#43.Weber leaf box template - Aj Concrete Pump
Browse 1,150 incredible Marijuana Leaf vectors, icons, clipart graphics, and backgrounds for royalty-free download from the creative contributors at ... 於 concretepumpsydney.com.au -
#44.Tomato yellow leaf curl virus alters the host preferences of its ...
Bemisia tabaci, the whitefly vector of Tomato yellow leaf curl virus (TYLCV), seriously reduces tomato production and quality. 於 www.nature.com -
#45.Leaf Logo High Resolution Stock Photography and Images
Green Power Energy Logo Design Element, Thunder Leaf Logo, Leaves icon vector, creative green leaf logo template, Eco icon green leaf. 於 www.alamy.com -
#46.3,000+ Free Leaf & Leaves Vectors - Pixabay
3,043 Free vector graphics of Leaf. Related Images: leavesflowerplantfloralframetreeflowersnaturepattern. Leaf vector images for download. All vector art is ... 於 pixabay.com -
#47.Compact Leaf Print Wallet - Blue - Woman - Parfois.com
Compact leaf print wallet. Zip fastening. Two inner card slots. Coin compartment with zip. Four outer pockets. Product Details. Main Material: 100 ... 於 www.parfois.com -
#48.Leaf Optical Properties - 第 341 頁 - Google 圖書結果
The choice of wavelengths or the leaf biochemical constituents may be ... notation: Y = Xβ + ε (11.31) where Y is an n x 1 column vector of concentrations, ... 於 books.google.com.tw -
#49.EFTOWEL Skateboards Japanese Green Overlap Bamboo Se ...
... Mail order cheap Leaf Vector 44 Outdoor Recreation Sports Outdoors EFTOWEL Skateboards Japanese Green Overlap Bamboo Leaf Vector Se Leaf,Bamboo,Vector ... 於 www.benesseresalute.net -
#50.Plant Diseases and Vectors: Ecology and Epidemiology
... 13 Rice orange leaf disease, vector of, 139 Rice penyakit habang, 108 Rice penyakit merah, 108 incidence of, 129- 130 vectors of, 137 Rice ragged stunt, ... 於 books.google.com.tw -
#51.Leaf Vector, 52000+ Leaf Graphic Resources for Free Download
You can download free Leaf vector in .AI and .EPS format. Thousands of new Leaf vector resources are added every day. 於 pngtree.com -
#52.Leaf Silhouette Vectors and Templates - FreePatternsArea
If you are looking for printable leaves template for crafting or leaf images for vector projects, these designs may be just for you. 於 www.freepatternsarea.com -
#53.The Veins of a Leaf: Revealing Nature's Mathematical System
Nature is a great architect, and the vascular network - or veins - of a leaf are key to its structure. Mathematical physicists at ... 於 www.pbs.org -
#54.Vector-Borne Diseases: Epidemiology and Control
Larvicidal activity of leaf extracts of Citrullus colocynthis against the vector mosquitoes 95% confidence Solvent Test organisms limits (ppm) ChiLC50 ... 於 books.google.com.tw -
#55.Leaf Logo Vectors Free Download - seeklogo
Leaf logo vectors. We have 163 free Leaf vector logos, logo templates and icons. You can download in .AI, .EPS, .CDR, .SVG, .PNG formats. 於 seeklogo.com -
#56.Vector Leaves on Behance
Vector Leaves for Photoshop & Illustrator. A collection of tropical leaf vectors in 3 different styles, perfect for adding clean & modern ... 於 www.behance.net -
#57.Leaf Vector Art, Icons, and Graphics for Free Download
Browse 146124 incredible Leaf vectors, icons, clipart graphics, and backgrounds for royalty-free download from the creative contributors at Vecteezy! 於 www.vecteezy.com -
#58.Deep feature based rice leaf disease identification using ...
In addition, the performance evaluation of 11 CNN models in transfer learning approach and deep feature plus support vector machine (SVM) was carried out. The ... 於 www.sciencedirect.com -
#59.11 Leaf Vector ideas | textures patterns, leaves vector, prints
Apr 24, 2014 - Explore Sunny Chang's board "Leaf Vector" on Pinterest. See more ideas about textures patterns, leaves vector, prints. 於 www.pinterest.com -
#60.Editable Leaves - Spring Leaf Template - Twinkl
A set of helpful editable Spring leaf templates, useful for a variety of activities. From classroom displays to creating family trees and more. 於 www.twinkl.co.uk -
#61.How to Make a leaf using vector graphics in Adobe Illustrator 5
This tutorial starts with a blank project and shows you how to use vector graphics to create a simple leaf in Adobe Illustrator 5. 於 adobe-illustrator.wonderhowto.com -
#62.How to Draw a Leaf | Vectornator Blog
Drawing with vectors is easier than you think! Here we will focus on leaves! Follow this step-by-step tutorial on how to draw a leaf in ... 於 www.vectornator.io -
#63.1,724,655 Leaf Vector Illustrations & Clip Art - iStock
Set vector silhouettes tropical leaves. Monochrome jungle exotic leaf philodendron, areca palm, royal fern, banana leaf. Illustration for summer tropical ... 於 www.istockphoto.com -
#64.Weber leaf box template - Cricket Pakistan
Browse 1,150 incredible Marijuana Leaf vectors, icons, clipart graphics, and backgrounds for royalty-free download from the creative contributors at ... 於 design.cricketpakistan.com.pk -
#65.Leaf Icons - 32,459 free icons - Flaticon
32459 leaf icons. Vector icons in SVG, PSD, PNG, EPS and ICON FONT. 於 www.flaticon.com -
#66.Vector Leaf Royalty-Free Stock Image - Storyblocks
Get Vector Leaf royalty-free stock image and other vectors, photos, and illustrations with your Storyblocks membership. 於 www.storyblocks.com -
#67.2103 leaves Vectors & Graphics to Download - Vexels
Leaves vector graphics to download in AI, SVG, JPG and PNG. New leaves designs everyday with Commercial licenses | Templates, logos, patterns & more. 於 www.vexels.com -
#68.Vector Leaves PNG Images, Free Transparent ... - kindpng
Discover 208 free Vector Leaves png images with transparent backgrounds. Search more hd transparent Vector Leaves image on KindPNG. 於 www.kindpng.com -
#69.Leaf Outdoor Throw Pillow - Opalhouse™ Designed With ...
Read reviews and buy Leaf Outdoor Throw Pillow - Opalhouse™ designed with Jungalow™ at Target. Choose from Same Day Delivery, Drive Up or Order Pickup. 於 www.target.com -
#70.Free leaf - Vector Art - Vector4Free
Free Vector Leaf - 45 royalty free vector graphics and clipart matching leaf. Sponsored Images by iStock. Watercolor autumn leaves · Vector leaf fall on an ... 於 www.vector4free.com -
#71.25 Free High Quality Vector Trees and Leaves For Designers
The below tree and leaf vector graphics are free to use however please check for commercial usage licenses.Enjoy. 於 designbeep.com -
#72.Leaf Vector Art & Graphics | freevector.com
Download Free Leaf Vectors and other types of leaf graphics and clipart at FreeVector.com! 於 www.freevector.com -
#73.Leaf vectors images graphic art designs - All-free-download.com
Leaf vectors images files in editable .ai .eps .svg format ✓ Premium quality ✓ Free for commercial use ✓ Free & easy download ✓ unlimit ✓ Update daily. 於 all-free-download.com -
#74.Nissan USA: Shop Online for Cars, Trucks, SUVs & Crossovers
Visit the official Nissan USA site to shop online for Nissan cars, trucks, crossovers, SUVs and vans. Explore vehicle features, pricing, offers and more. 於 www.nissanusa.com