car driving class的問題,透過圖書和論文來找解法和答案更準確安心。 我們找到下列包括價格和評價等資訊懶人包
car driving class的問題,我們搜遍了碩博士論文和台灣出版的書籍,推薦Taseer, Shahbaz寫的 Lost to the World 和Morgan, Sophie的 Miss Fortune都 可以從中找到所需的評價。
這兩本書分別來自 和所出版 。
朝陽科技大學 工業工程與管理系 林宏達所指導 鄭丞凱的 電腦視覺技術應用於手工具組裝之零件瑕疵檢驗 (2021),提出car driving class關鍵因素是什麼,來自於自動化檢驗、手工具組裝、瑕疵檢驗、R-CNN網路模式。
而第二篇論文國立中正大學 電機工程研究所 賴文能所指導 洪金利的 基於單影像之六自由度物體姿態估測 (2021),提出因為有 的重點而找出了 car driving class的解答。
Lost to the World
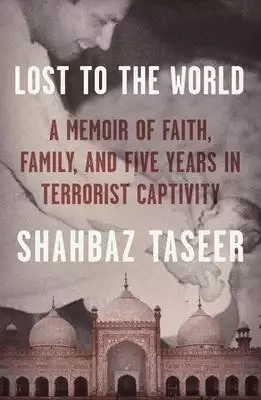
為了解決car driving class 的問題,作者Taseer, Shahbaz 這樣論述:
Shahbaz Taseer’s memoir of his five-year-long captivity at the hands of the Islamic Movement of Uzbekistan.In late August 2011, Shahbaz Taseer was driving to his office in Lahore when he was dragged from his car at gunpoint and kidnapped by members of the Islamic Movement of Uzbekistan (IMU), a T
aliban-affiliated Uzbek terrorist group. Shahbaz’s father, the late Pakistani governor, had recently been assassinated. His crime: speaking in support of a Christian woman who had been accused of blasphemy and sentenced to death. Though Taseer himself wasn’t much interested in politics, he was somew
hat of a public figure, and he represented a more tolerant, internationally connected Pakistan that the IMU despised. What followed was nearly five years of torture and harrowing danger while Taseer was held captive, his fate determined by the infighting of the IMU, the Taliban, and ISIS. Lost to th
e World is his memoir of that time--a story of extraordinary sorrow but also of goodness and faith. While deeply dramatic, this tale is also comedic; for Taseer, humor, as much as the Koran, provided a light by which to see his own humanity, even under the most inhumane conditions, and to find a way
back to his family. In a time when Western leaders use fear-mongering rhetoric to paint all followers of Islam as dangerous fundamentalists, Lost to the World illustrates the chasm between Muslim terrorists and ordinary Muslim citizens, and how terrorist organizations gain strength from the war on
terror.
car driving class進入發燒排行的影片
เปิดตัวครั้งแรกในโลก พร้อมราคา The All-New Mitsubishi Outlander 2021-2022 World Premiere มิตซูบิชิ เอาท์แลนเดอร์ โฉมใหม่ล่าสุด มี 3 แถว 7 ที่้นั่ง ทำตลาดในอเมริกาเหนือ ก่อนมาขายในไทย
Mitsubishi Motors Corporation ประกาศเปิดตัว All-New outlander โฉมใหม่ เจนเนอเรชั่นที่ 4 รุ่นปี 2022 รถครอสโอเวอร์รุ่น flagship ของบริษัท ผ่านทาง Amazon Livestream เป็นครั้งแรก โดยมาพร้อมดีไซน์ใหม่ คุณภาพระดับพรีเมี่ยม สมรรถนะที่เหนือชั้น และเทคโนโลยีที่ล้ำสมัย ที่คุณสามารถคาดหวังได้ จากรถยนต์ของ Mitsubishi พร้อมเริ่มจำหน่ายในตลาดอเมริกาเหนือ เป็นที่แรกในโลก ในราคาเริ่มต้น 25,795 เหรียญสหรัฐ หรือราว 773,000 บาท ในเดือนเมษายน ปี 2021 นี้ ก่อนที่จะมีการทำตลาดทั่วโลก หลังจากนั้น
FRANKLIN, Tenn. – As part of a game-changing collaboration with Amazon, MITSUBISHI MOTORS CORPORATION (MMC) today revealed the all-new 2022 OUTLANDER crossover SUV via livestream, the first vehicle to ever debut on Amazon Live.2 All-new from the wheels up, the 2022 Outlander features a new design direction for both this vehicle and the brand, plus the premium quality, rugged performance and innovative technology expected of a Mitsubishi Motors vehicle.
The flagship of the Mitsubishi Motors line, it is reimagined and reinvented in every way, and is the best-equipped, most thoughtfully engineered vehicle the company has ever developed. Outlander gears up for sale in North America first in April 2021, with other global markets to follow.
With a U.S. Manufacturer's Suggested Retail Price starting at $25,7951, the all-new 2022 Mitsubishi Outlander delivers the equipment, quality and lasting value that Mitsubishi customers have come to expect of the brand. Full pricing and packaging details will be made available at a later date.
"Based on the product concept 'I-Fu-Do-Do,' which means authentic and majestic in Japanese, the all-new OUTLANDER has been crafted into a reliable SUV with significantly upgraded styling, road performance, and a high-quality feel to satisfy the needs of customers who want to expand their horizons and take on challenges of every kind," said Takao Kato, chief executive officer of MMC. "With the launch of the all-new OUTLANDER, we will first expand our sales in the North American market and then aim for global growth."
The Outlander was first launched in North America in 2002, and this new model is the fourth generation to be sold.
Styling debuts the brand's next generation Dynamic Shield front face and design language, with muscular fenders, bold proportions and available large-diameter 20-inch wheels. Inside, Outlander is a quiet and serene space, showcasing quality and convenience through class-above materials, seating for seven in the segment's only standard-equipment third-row, available 12.3-inch digital instrument cluster and 9-inch center screen, and also newly available wireless smartphone charging capability with Android Auto3 and wireless Apple CarPlay.4
The engineering underpinnings are also all-new. Partnered with a newly developed platform and 2.5L four-cylinder engine, Mitsubishi's rally-derived Super All-Wheel Control5 system provides unmatched confidence for drivers in all environments. The newly developed drive mode selector allows performance and grip to be tailored to the conditions through six distinct settings, increasing on-road and off-pavement performance. Even two-wheel drive models are fitted with the drive-mode selector, offering five distinct modes in this setup, to help drivers feel more confident in all driving conditions.
Standard equipment on the 2022 Outlander includes 11 airbags6, three rows of seats, myriad storage locations, USB-A and USB-C charge ports and 18-inch wheels.
Depending on trim level, the 2022 Outlander can be fitted with 20-inch wheels, Mitsubishi's MI-PILOT Assist driver assistance system with adaptive cruise control and lane-keep assist7, semi-aniline leather seating, integrated navigation using what3words technology, a windshield-display 10.8-inch full-color Head-Up Display (HUD), Mitsubishi's industry-leading Mitsubishi Connect smart-car system, and a 10-speaker BOSE® audio system.8
Mitsubishi Motors North America is in the midst of introducing a full showroom of redesigned, reengineered or all-new vehicles, and the 2022 Outlander is the culmination of that program. This much-anticipated vehicle is here, and the game-changing launch is well under way. The all-new 2022 Mitsubishi Outlander is set to break boundaries, reset expectations and demand attention.
電腦視覺技術應用於手工具組裝之零件瑕疵檢驗
為了解決car driving class 的問題,作者鄭丞凱 這樣論述:
目錄摘要 IAbstract II目錄 IV圖目錄 VII表目錄 XII第一章 緒論 I1.1 棘輪扳手與零件介紹 21.2 棘輪扳手組裝流程 51.3 棘輪扳手組裝異常類型與瑕疵種類 71.4 棘輪扳手組裝之現行檢驗方式 181.5 研究動機與目的 191.6 論文架構 21第二章 文獻探討 222.1 自動化視覺檢測 222.2 組裝異常檢測 232.3 物件特徵比對 252.4 類神經網路模型 262.4.1 卷積神經網路(Convolutional Neural Network, CNN) 262.4.2 YOLOV4 (You O
nly Look Once)網路模型 272.4.3 基於區域的卷積神經網路(Region With CNN, R-CNN) 282.4.4 快速的基於區域的卷積神經網路(Fast R-CNN) 292.4.5 更快速的基於區域的卷積神經網路(Faster R-CNN) 302.4.6 基於遮罩的區域卷積神經網路(Mask R-CNN) 32第三章 研究方法相關原理 363.1 工件影像濾波 363.2 常見之物件偵測分類器 373.2.1 CNN網路模型 383.2.2 YOLO系列模型 393.2.3 R-CNN系列模型 40第四章 研究流程與技術應用 514.
1 工件影像拍攝 534.2 影像之ROI區域擷取 544.3 ROI影像之濾波處理 554.4 工件組裝異常之瑕疵種類特徵擷取 574.5 工件組裝異常類型之瑕疵種類的分類 604.5.1 物件候選區域選擇 614.5.2 CNN網路模式之特徵提取 624.5.3支援向量機的瑕疵分類 634.5.4 可疑瑕疵區域的邊界框回歸 644.5.5 瑕疵種類分類結果輸出 664.6 工件組裝異常類型之瑕疵種類的分類績效混淆矩陣 67第五章 實驗結果與分析 695.1 樣本影像說明 695.2 組裝異常之瑕疵檢測系統之發展 705.3 組裝異常類型之瑕疵種類分類績效指標
715.4 組裝異常之瑕疵檢測系統之R-CNN網路模型之參數設定 725.4.1 網路模型之學習率參數設定 745.4.2 網路模型之訓練批量參數設定 765.4.3 網路模型之優化器類型選擇 785.4.4 網路模型之訓練次數參數設定 805.4.5 網路模型避免過度擬合之判斷設定 825.5 組裝異常檢測之分類績效評估與比較 845.5.1 R-CNN系列模型比較 845.5.2 R-CNN系列模式與YOLOV4之檢測績效比較 895.6 敏感度分析 955.6.1 ROI區域大小對檢測效益之影響 965.6.2 影像亮度的變化對檢測績效之影響 975.6.3
工件擺放方式對檢測績效之影響 995.6.4 工件表面油漬量對檢驗績效之影響 1035.6.5 工件輸送帶速度對檢測績效之影響 1085.6.6 棘輪扳手單一分類器檢驗模型選擇 1135.6.7 同態濾波對檢測效益之影響 115第六章 結論與後續研究方向 1186.1 結論 1186.2 未來研究方向 119參考文獻 122表目錄表1 市售主要棘輪扳手之英制與公制規格 3表 2 1/2”36T棘輪扳手各組裝站之零件表 4表3 棘輪扳手組裝之各工作站的工作內容說明表 5表4 棘輪扳手組裝時可能產生的組裝異常類型說明彙整表 8表5 棘輪扳手組裝過程
可能的組裝異常類型與瑕疵種類彙整表 9表6 缺件組裝異常之瑕疵種類影像彙整表 14表7 錯置組裝異常之瑕疵種類影像彙整表 15表8 異物組裝異常之瑕疵種類影像彙整表 16表9 餘件組裝異常之瑕疵種類影像彙整表 17表10 取像限制說明表 21表11 本研究與物件偵測相關文獻比較表 35表12 本研究使用之網路模型比較表 48表13 本研究目前使用之遮罩與影像面積之比較表(單位:pixel) 55表14 灰階影像與濾波後影像之平均值及標準差比較表 57表15 以影像張數為基礎之棘輪扳手分類混淆矩陣示意表 68表16 棘輪扳手檢驗結果之混淆矩陣示意表
68表17 本研究組裝第一站之檢測樣本影像數量 73表18 本研究組裝第二站之檢測樣本影像數量 74表19 本研究組裝第三站之檢測樣本影像數量 74表20 採用不同學習率之檢測效益結果比較 75表21 採用不同訓練批量之檢測效益結果比較 77表22 本研究探討之三種優化演算法優缺點比較 79表23 採用不同網路模型優化器之檢測效益結果比較 79表24 採用不同網路模型訓練次數之檢測效益結果比較 81表25 R-CNN網路模型之預設值與較佳參數設定之比較表 84表26 第一站大樣本異常類型之瑕疵種類檢驗模型效益彙整表 86表27 第二站大樣本異常類型之瑕
疵種類檢驗模型效益彙整表 87表28 第三站大樣本異常類型之瑕疵種類檢驗模型效益彙整表 88表29 本研究組裝工作站之較佳網路模型效益彙整表 89表30 第一站較佳模型與YOLOV4之檢測效益比較表 90表31 第二站較佳模型與YOLOV4之檢測效益比較表 91表32 第三站較佳模型與YOLOV4之檢測效益比較表 92表33 第一站各網路模型之檢測時間彙整表(單位:秒) 93表34 第二站各網路模型之檢測時間彙整表(單位:秒) 93表35 第三站各網路模型之檢測時間彙整表(單位:秒) 93表36 採用不同遮罩大小之檢測效益結果比較 96表37 採用拍攝光
線強度之檢測效益結果比較 98表38 工件偏移角度之影像數量彙整表 101表39 棘輪扳手不同擺放角度之檢測效益比較表 101表40 ROI區域與油漬量之影像面積比較表(單位:pixel) 104表41 塗抹不同程度潤滑油之檢測效益比較表 106表42 靜態與動態拍攝之差異比較表 109表43 不同輸送帶速度之影像檢測效率 111表44 棘輪扳手動態視覺檢測系統之檢測效益比較表 112表45 棘輪扳手各站模型之正確分類率比較表 114表46 灰階影像與濾波後影像之影像像素比較表 116表47 第一站各模型有無經同態濾波處理之檢測效益彙整表 117圖目錄
圖1 市售棘輪扳手常見之產品銷售方式 I圖2 棘輪扳手的使用說明 2圖3 完成組裝之1/2” 36T棘輪扳手 3圖4 1/2”扭力頭寬度規格標示 3圖5 1/2”36T棘輪扳手之內部結構 3圖6 36T扭力頭實體圖(圓圈標示處為該零件之齒輪) 4圖7 葫蘆柄各組裝站之零件彙整 6圖8 棘輪扳手之組裝異常類型與瑕疵種類關係彙整圖 10圖9 第一站經組裝後各種可能的缺件組裝異常結果 11圖10 第二站經組裝後各種可能的缺件組裝異常結果 12圖11 第三站經組裝後各種可能的缺件組裝異常結果 13圖12 棘輪扳手檢驗實體圖 19圖13 同態濾波器的運算
流程 37圖14 CNN網路架構示意圖 38圖15 卷積方法示意圖 39圖16 池化運算示意圖 39圖17 YOLOV4網路架構示意圖 40圖18 R-CNN網路架構示意圖 41圖19 Fast R-CNN網路架構示意圖 43圖20 ROI pooling運算示意圖 44圖21 Faster R-CNN網路架構示意圖 45圖22 RPN運算示意圖 46圖23 Mask R-CNN網路架構示意 47圖24 研究方法流程圖 52圖25 本研究現階段使用之數量與零件 53圖26 本研究之硬體設備架設示意圖 53圖27 本研究前處理之影像平均值與
標準差 54圖28 本研究使用之五種遮罩大小 55圖29 使用同態濾波濾除拍攝時造成反光之像素變化 56圖30 灰階影像與濾波後影像之平均值及標準差曲線圖 57圖31 光源控制器數值下灰階影像與濾波後影像標準差比較表 57圖32 使用Matlab軟體內建之Image Labeler工具箱進行人工標...58圖33 完成標註之邊界框資訊 58圖34 棘輪扳手組裝製程中第一組裝站使用R-CNN網路模式之圖像標註流程圖 59圖35 第一站缺件檢驗之R-CNN網路架構的訓練程序 60圖36 R-CNN模型檢驗流程圖 61圖37 候選區域選擇示意圖 62圖38
特徵提取流程圖 63圖39 邊界框回歸原理示意圖 65圖40 邊界框回歸運算可能發生之失效結果 66圖41 瑕疵種類分類結果示意圖 67圖42 運用R-CNN網路模型之棘輪扳手檢驗辨識系統測試程序 67圖43 本研究之實驗架構圖 69圖44 本研究影像拍攝之設備圖 70圖45 本研究所開發之使用者介面 71圖46 不同學習率之檢出績效評估ROC曲線圖 75圖47 不同學習率之正確分類率折線圖 76圖48 不同訓練批量之檢出績效評估ROC曲線圖 77圖49 不同訓練批量之正確分類率折線圖 77圖50 不同網路模型優化器之檢出績效評估ROC曲線圖
80圖51 不同網路模型優化器之正確分類率折線圖 80圖52 不同訓練次數之檢出績效評估ROC曲線圖 82圖53 不同訓練次數之正確分類率折線圖 82圖54 本研究使用R-CNN網路模型之訓練資料損失曲線圖 83圖55 過擬合現象示意圖 83圖56 第一站R-CNN系列模型之ROC曲線圖 86圖57 第一站R-CNN系列模型之績效指標曲線圖 86圖58 第二站R-CNN系列模型之ROC曲線圖 87圖59 第二站R-CNN系列模型之績效指標曲線圖 87圖60 第三站R-CNN系列模型之ROC曲線圖 88圖61 第三站R-CNN系列模型之績效指標曲線圖
88圖62 第一站R-CNN系列較佳模型與YOLOV4之ROC曲線圖 90圖63 第一站R-CNN系列較佳模型與YOLOV4之績效指標曲線圖 90圖64 第二站R-CNN系列較佳模型與YOLOV4之ROC曲線圖 91圖65 第二站R-CNN系列較佳模型與YOLOV4之績效指標曲線圖 91圖66 第三站R-CNN系列較佳模型與YOLOV4之ROC曲線圖 92圖67 第三站R-CNN系列較佳模型與YOLOV4之績效指標曲線圖 92圖68 R-CNN系列模型與YOLOV4之總訓練時間曲線圖 94圖69 R-CNN系列模型與YOLOV4之總測試時間曲線圖 94圖70
R-CNN系列模型與YOLOV4之單位影像測試時間曲線圖 94圖71 各站R-CNN系列較佳模型與YOLOV4之正確分辨率直方圖 95圖72 使用不同遮罩大小之棘輪扳手檢出績效評估ROC曲線 97圖73 使用不同遮罩大小之棘輪扳手正確分類率折線圖 97圖74 採用不同亮度拍攝棘輪扳手之檢出率與誤判率ROC曲線 98圖75 採用不同亮度拍攝棘輪扳手之正確分類率折線圖 98圖76 工件擺放方向示意圖 99圖77 原始影像之各角度擺放情況 100圖78 原始影像加入遮罩後各角度擺放情況 100圖79 棘輪扳手正向擺設角度之檢出績效評估ROC曲線 102圖80
棘輪扳手負向擺設角度之檢出績效評估ROC曲線 102圖81 棘輪扳手擺設角度之正確分類率折線圖 103圖82 第一站塗抹不同程度潤滑油之比較圖 104圖83 第二站塗抹不同程度潤滑油之比較圖 104圖84 第一站塗抹不同程度之潤滑油後加上遮罩之比較圖 105圖85 第二站塗抹不同程度之潤滑油後加上遮罩之比較圖 105圖86 第一站塗抹不同程度潤滑油之檢出績效評估ROC曲線圖 106圖87 第一站塗抹不同程度潤滑油之正確分類率折線圖 107圖88 第二站塗抹不同程度潤滑油之檢出績效評估ROC曲線圖 107圖89 第二站塗抹不同程度潤滑油之正確分類率折線圖 1
07圖90 棘輪扳手動態視覺檢測系統運作示意圖 108圖91 棘輪扳手動態視覺檢測系統硬體架設實體圖 110圖92 動態視覺檢測系統中不同輸送帶速度所拍攝之原始影像 110圖93 動態視覺檢測系統中不同輸送帶速度所拍攝之前處理影像 111圖94 棘輪扳手動態視覺檢測系統之ROC曲線圖 112圖95 棘輪扳手動態視覺檢測系統之正確分類率曲線圖 113圖96 棘輪扳手各站模型之正確分類率直方圖 114圖97 棘輪扳手各站模型之檢測時間直方圖 115圖98 有無經同態濾波處理對各模型之正確分類率直方圖 117圖99 有無經同態濾波處理對各模型之績效指標折線圖 11
7
Miss Fortune
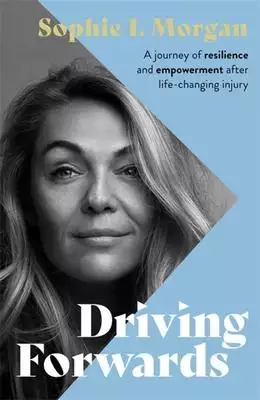
為了解決car driving class 的問題,作者Morgan, Sophie 這樣論述:
’A book that’ll change your perspective on life. You’ll not be able to put it down.’ Fearne Cotton’Everyone should read this book. Sophie Morgan is the epitome of grit and determination. Her writing is thought provoking, honest and in parts hilarious.’ Katie Piper OBE’Wrenchingly honest...eye-ope
ning and deeply moving. *****’ Mail on SundayAs seen on ’Living Wild; How to Change your Life’ a two-part prime-time series on Channel 4, Loose Women and The Great Celebrity Bake Off for SU2C On the precipice of starting her adult life, aged eighteen, Sophie, a rebellious and incorrigible wild child
, crashed her car and was instantly paralysed from the chest down. Rushed to hospital, everything she had dreamed for her life was instantly forgotten and her journey to rediscover herself and build a different life began. But being told she would never walk again would come to be the least of her c
oncerns. Over the next eighteen years, as she strived to come to terms with the change in her body, her relationships were put to the test; she has had to learn to cope with the many unexpected and unpredictable setbacks of living with paralysis; she has had to overcome her own and other people’s pe
rceptions of disability and explore the limits of her abilities, all whilst searching for love, acceptance, meaning, identity, and purpose. Driving Forwards is a remarkable and powerful memoir, detailing Sophie’s life-changing injury, her recovery, and her life since. Strikingly honest, her story is
unusual and yet relatable, inspiring us to see how adversity can be channelled into opportunity and how ongoing resilience can ultimately lead to empowerment. ’Raw, life affirming and gorgeously written - this book is filled with extraordinary honesty, courage and warmth. Sophie’s words will make u
s all braver and more hopeful.’ Daisy Buchanan’A truly astonishing read about the power of never giving up.’ Sun’F***king hell!! This book is absolutely brilliant . . . One of the best memoirs I’ve ever read. Honest and so blooming human, it’s fantastic.’ Kathy Burke
基於單影像之六自由度物體姿態估測
為了解決car driving class 的問題,作者洪金利 這樣論述:
Dealing with the object pose estimation from a single RGB image is very challenging since 6 degree-of-freedom (6DoF) parameters have to be predicted without using the spatial depth information. Since direct regression of the pose parameters by using the deep neural network was reportedly poor and t
hen attaching with the refinement module to improve the accuracy causes much time consumption, in this work, we propose several techniques of top-down or bottom-up approaches to predict indirect feature maps instead from which single or multiple object poses can be recovered by using sophisticated p
ost-processing algorithms.Since there are four possible scenarios where single/multiple objects in the same/different classes can appear in the image, the corresponding output feature maps are predicted differently. For a single object scenario, unit-vector fields are predicted. These features are c
omposed of many unit-vectors pointing from pixels within the object mask to the pre-defined 2D object keypoints where their corresponding 3D object keypoints are distributed optimally on the 3D object surface based on the keypoint distances and object surface curvatures. From some pairs of the predi
cted unit-vectors, 2D projected keypoints can be voted and determined, so that PnP algorithm can be applied to estimate the pose. To deal with multiple objects even in the same or different classes, sufficient and informative output feature maps need to be predicted. Different from object keypoints,
6D coordinate maps which form the main features can be considered as a bunch of 3D point clouds for pose parameter calculation when their 2D-3D correspondences are also established. 6D coordinate maps contains two parts: front- and rear-view 3D coordinate maps. 3D coordinate map is actually a 2D ma
p where each pixel records 3D coordinates of a point in the object CAD model which projects to that 2D pixel location. Via 3D/6D coordinate maps, instance 2D-3D correspondences of a large point set can be built and PnP algorithm combined with RANSAC scheme to overcome the outliers or noise can be us
ed to estimate multiple object poses. Even though in this case, 2D object keypoints can no longer be used to estimate multiple poses, they can be defined as single/multiple reference points for identifying all object instance masks even in the presence of heavy occlusion. We are also interested in o
vercoming some problems related to the missing information and symmetry ambiguity encountered when generating the ground truth of 6D coordinate maps.Our studies show that our single pose estimation method using unit-vector fields can achieve an outstanding accuracy if compared to other top-down stat
e-of-the-art methods without including refinement modules. It has a good algorithm to identify the designated object keypoints from which the predicted feature maps are trained with the effective loss functions, but it has a slower inference speed when multiple object poses are taken into considerat
ion. On the other hand, our 6D coordinate maps, combining with the information from two opposite views, are capable of providing more constraints for network optimization and hence helpful for pose estimation accuracy. Our methods using 6D coordinate maps can achieve great performances if compared t
o other multiple object pose estimation methods.