car driving learning的問題,透過圖書和論文來找解法和答案更準確安心。 我們找到下列包括價格和評價等資訊懶人包
car driving learning的問題,我們搜遍了碩博士論文和台灣出版的書籍,推薦Miller, John,McCormack, Susan寫的 Practical Teaching Skills for Driving Instructors: Developing Your Client-Centred Learning and Coaching Skills 和Miller, John,McCormack, Susan的 Practical Teaching Skills for Driving Instructors: Developing Your Client-Centred Learning and Coaching Skills都 可以從中找到所需的評價。
這兩本書分別來自 和所出版 。
國立中正大學 電機工程研究所 余英豪所指導 徐雋航的 基於語意之輪廓表示法及全連結捲積類神經網路之單晶片多車輛辨識系統 (2021),提出car driving learning關鍵因素是什麼,來自於車輛辨識、語意之輪廓表示法、類神經網路、車距檢測。
而第二篇論文國立中正大學 電機工程研究所 余英豪所指導 廖國欽的 基於FPGA單晶片及像素趨勢車道線檢測法實現車道線感測系統之研究 (2021),提出因為有 自動駕駛、車道線辨識、即時處理系統、先進駕駛輔助系統、線性回歸的重點而找出了 car driving learning的解答。
Practical Teaching Skills for Driving Instructors: Developing Your Client-Centred Learning and Coaching Skills
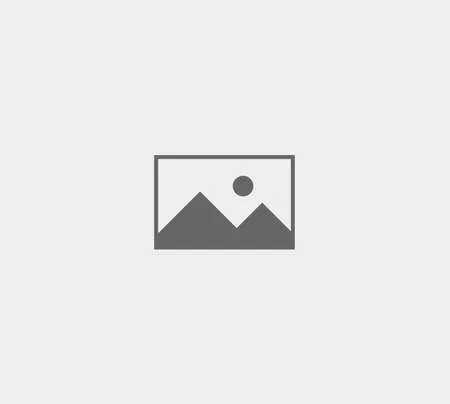
為了解決car driving learning 的問題,作者Miller, John,McCormack, Susan 這樣論述:
John Miller has been involved in the driver training industry for over 35 years, based in Chichester, UK. He is a qualified and experienced instructor trainer and LGV instructor. For many years he ran his own driving school for car and lorry drivers in Chichester, together with an instructor trainin
g facility. He is now a consultant to the driver training industry. Susan McCormack is Managing Director of Tri-Coaching Partnership, which focuses on training and developing Approved Driving Instructors (ADIs), based in East Anglia, UK. A regular contributor to industry media, she has created sever
al training courses, including the Pearson-accredited BTEC Level 4 in Coaching for Driver Development.
car driving learning進入發燒排行的影片
✅ Thank you for watching the video: ❤️
►Other videos here: https://www.youtube.com/playlist?list=PLoR07j8u5LFBw30MAQD7UfVWsNnkp5nlQ
*Watch in UltraHD #Shorts #VehicleVirgins #COOLVEHICLETV
? The GirlsCoolCarsTV of Youtube : https://www.youtube.com/channel/UCSVcIYa_rBYxon3V4XBPXwA?sub_confirmation=1
▬▬▬▬▬▬▬▬▬▬▬▬▬▬▬
*Description: https://www.youtube.com/watch?v=CcO4OGoCoHM&list=PLoR07j8u5LFDBft0D7nBP25ebD08X--h6
✔ Become a member to enjoy the perks! https://www.youtube.com/channel/UClI0o1LN8UbODMFhw4Qy8SQ/join
✔ 100.000 SUB PLEASE: https://bit.ly/3jhJpVC
✔ Don't forget Share, Like, Comment this video.
? The GIRLS COOL CARS TV of Youtube: https://www.youtube.com/channel/UCSVcIYa_rBYxon3V4XBPXwA?sub_confirmation=1
? The COOL VEHICLE TV of Youtube: https://www.youtube.com/channel/UCzRTWU_uLjfEsET8vRSDgDQ?sub_confirmation=1
⚡️ For more videos and articles visit: https://bit.ly/3cPpc7x
❤️ Thanks for your support !!
▬▬▬▬▬▬▬▬▬▬▬▬▬▬▬
? MY PLAYLISTS:
** Vehicle Virgins: https://www.youtube.com/playlist?list=PLoR07j8u5LFBw30MAQD7UfVWsNnkp5nlQ
** Pedal Vamp Girls Cool Cars TV: https://www.youtube.com/playlist?list=PLoR07j8u5LFDBft0D7nBP25ebD08X--h6
** Fun Driving Lesson: https://www.youtube.com/playlist?list=PLwdz5NnxN6gicUNEWQokjWQEZvyjyATuo
** Learn To Drive a Car: https://www.youtube.com/playlist?list=PLwdz5NnxN6gjZZazdZb8tDGhYM6aM4ceI
** Pedal Vamp Cool Lady Vehicle: https://www.youtube.com/playlist?list=PLwdz5NnxN6ghJ4nNOMYsZ-tGaCr6HLJY8
✅ THANKS for WATCHING and HAVE A NICE DAY!
最新おはよう寺ちゃん: https://www.youtube.com/playlist?list=PLA6HuNxO0vlPb56dzxiCAH_ebXnqBundy
Twitter: https://twitter.com/Japanesenews247
ここで私をサポートしてください:https://www.youtube.com/channel/UC4ikJtUMsc2c8XhuKLlKMWg/join
#おはよう寺ちゃん活動中
#寺島尚正 #篠原常一郎
基於語意之輪廓表示法及全連結捲積類神經網路之單晶片多車輛辨識系統
為了解決car driving learning 的問題,作者徐雋航 這樣論述:
鑒於現今智慧車輛發展迅速,前方車輛辨識及車距檢測為先進駕駛輔助系統 (Advanced Driver Assistance Systems, ADAS) 設計中相當重要的一環,此項技術通常藉由攝影鏡頭擷取前方影像,並透過影像辨識技術來判斷前方是否存在車輛、障礙物等等,進而控制車輛減速以保持安全距離。而這些複雜的圖形辨識技術往往需要透過高功耗之大型運算系統來實現,並且,若將傳統電腦安裝於車內常需要克服體積過大、耐震性不佳等缺點。因此,本研究專注於如何將車輛辨識及車距檢測演算法實現於單晶片,以達到高性能、低功耗,以及體積小之目的。為實現前方車輛辨識及車距檢測,本研究透過單一彩色相機模組收集前方影
像資訊,並於單一現場可程式邏輯閘陣列 (Field Programmable Gate Array, FPGA) 晶片中以最精簡之硬體電路實現白平衡 (White Balance)、影像對比度強化技術 (Image Contrast Technique)、物體邊緣檢測、利用基於模糊語意影像描述 (Semantics-based Vague Image Representation, SVIR) 改良之基於語義之輪廓表示法 (Semantic-based Contour Representation, SCR) 特徵表達物體、再透過不同的卷積核 (Convolution Kernel) 重釋SC
R特徵並交由全連接類神經網路(Fully Connected Neural Network, FCN) 進行車輛辨識。最後,以多個邊界框 (Bounding Box) 同時檢測前方多台車輛,達到單頁多目標辨識 (Single Shot MultiBox Detector,SSD) 之功能,而邊界框之座標可以透視法 (Perspective View) 計算前車相對距離。根據本研究之實驗結果,在相機以每秒90張影像攝影速度以及影像解析度在640×480像素的條件下,本研究僅須3.61us即可完成單台車輛辨識,車輛辨識率可達到94%,且車輛與非車輛至少保持38%以上之分離度,有效減少感測錯誤的情況
發生。因此,實現一真正高性能、低功耗以及體積小之前方車輛辨識晶片。
Practical Teaching Skills for Driving Instructors: Developing Your Client-Centred Learning and Coaching Skills
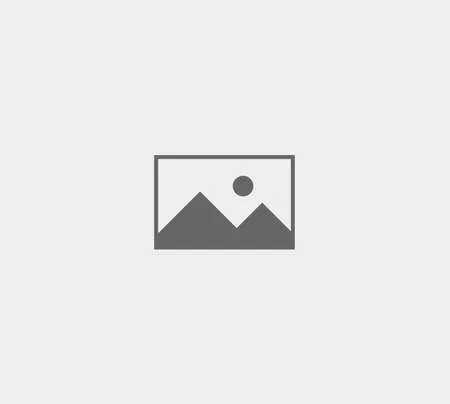
為了解決car driving learning 的問題,作者Miller, John,McCormack, Susan 這樣論述:
John Miller has been involved in the driver training industry for over 35 years, based in Chichester, UK. He is a qualified and experienced instructor trainer and LGV instructor. For many years he ran his own driving school for car and lorry drivers in Chichester, together with an instructor trainin
g facility. He is now a consultant to the driver training industry. Susan McCormack is Managing Director of Tri-Coaching Partnership, which focuses on training and developing Approved Driving Instructors (ADIs), based in East Anglia, UK. A regular contributor to industry media, she has created sever
al training courses, including the Pearson-accredited BTEC Level 4 in Coaching for Driver Development.
基於FPGA單晶片及像素趨勢車道線檢測法實現車道線感測系統之研究
為了解決car driving learning 的問題,作者廖國欽 這樣論述:
車輛自動駕駛系統目前主要是由自動跟車 (Adaptive Cruise Control, ACC) 以及車道偏離警示 (Lane Departure Warning System, LDWS) 兩大系統所組成。然而,自動跟車系統在實現過程中,由於必須藉由前方車輛實現車輛跟隨功能,因此若無前方車輛時則無法實現此功能。反觀車道偏離警示系統是依據車道線軌跡來幫助車輛保持於車道內,因此具備較高實用性。在此,本研究特別針對車道感測進行研究。由於傳統的車道線感測必須仰賴高效率的電腦才能有效地完成運算,為了克服傳統車道線辨識的缺點,本研究專注於如何將車道線辨識演算法簡化,並實現在單晶片上,達到低功耗之目的
。本研究以單一數位相機及單一現場可程式邏輯閘陣列 (Field Programmable Gate Array, FPGA) 實線以精簡之硬體電路達到即時於白天及黃昏情況下進行車道線辨識。透過像素趨勢車道檢測法 (Pixel Trend Lane Detection, PTLD) 擷取特徵,並將所得之車道位置利用線性回歸 (Linear Regression, LR) 決定車道線的軌跡,再透過左右車道回歸線取得車道的中心線,藉此引導車輛穩定行駛於車道中。另外,本研究還搭配語音辨識擴充模組 (DFR0177 Voice Recognition) 來辨識由Google Map路線規劃所傳出的語音指
令。根據辨識的結果,輸出行車指令給FPGA,以此決定車輛轉彎或直線行車路線模式。根據本研究之實驗結果,在使用每秒90張畫面播放速度以及640×480影像解析度情況下,只需11 ms即可擷取車道線特徵。而由左右車道線線性回歸決定出的中心線與實際影像中的中心線,誤差僅在5個像素以內。故本研究不管在運算速度以及準確度上均符合實際運用需求,未來可以有效幫助車輛穩定行駛於車道,達成自動駕駛之目的。