Component vector的問題,透過圖書和論文來找解法和答案更準確安心。 我們找到下列包括價格和評價等資訊懶人包
Component vector的問題,我們搜遍了碩博士論文和台灣出版的書籍,推薦Christensen, Ronald寫的 Plane Answers to Complex Questions: The Theory of Linear Models 和Nokeri, Tshepo Chris的 Data Science Revealed: With Feature Engineering, Data Visualization, Pipeline Development, and Hyperparameter Tuning都 可以從中找到所需的評價。
另外網站Selecting separate vector components - Blueprint也說明:Hi I'm trying to get the separate x, y, z components from a vector so can adjust the x value (making it negative) to then set those for the ...
這兩本書分別來自 和所出版 。
國立勤益科技大學 機械工程系 黃智勇所指導 許志安的 機械學習分類演算法在線性致動器缺陷元件檢測之應用 (2021),提出Component vector關鍵因素是什麼,來自於線性致動器、缺陷檢測、主成分分析、支持向量機、K-近鄰演算法。
而第二篇論文國立中正大學 會計與資訊科技碩士在職專班 許育峯所指導 洪郁翔的 一個植基於特徵選取與樣本選取技術的自動選股模型 (2021),提出因為有 自動選股模型、投資策略、分群演算法、特徵選取、樣本選取的重點而找出了 Component vector的解答。
最後網站POV-Ray: Documentation: 2.2.1.4 Vector Expressions則補充:Unless otherwise noted, all 2, 4 or 5 component vectors work just like 3D vectors but they have a different number of components. The syntax for combining ...
Plane Answers to Complex Questions: The Theory of Linear Models

為了解決Component vector 的問題,作者Christensen, Ronald 這樣論述:
This textbook provides a wide-ranging introduction to the use and theory of linear models for analyzing data. The author’s emphasis is on providing a unified treatment of linear models, including analysis of variance models and regression models, based on projections, orthogonality, and other vec
tor space ideas. Every chapter comes with numerous exercises and examples that make it ideal for a graduate-level course. All of the standard topics are covered in depth: ANOVA, estimation including Bayesian estimation, hypothesis testing, multiple comparisons, regression analysis, and experimental
design models. In addition, the book covers topics that are not usually treated at this level, but which are important in their own right: balanced incomplete block designs, testing for lack of fit, testing for independence, models with singular covariance matrices, variance component estimation, be
st linear and best linear unbiased prediction, collinearity, and variable selection. This new edition includes a more extensive discussion of best prediction and associated ideas of R2, as well as new sections on inner products and perpendicular projections for more general spaces and Milliken and G
raybill’s generalization of Tukey’s one degree of freedom for nonadditivity test.
Component vector進入發燒排行的影片
Section II Force and Motion
2.2 Force and Motion
Addition and resolution of forces
機械學習分類演算法在線性致動器缺陷元件檢測之應用
為了解決Component vector 的問題,作者許志安 這樣論述:
將線性滑軌與精密滾珠導螺桿的功能整合在單一組件的線性致動器,因兼具高剛性與行程精度,常應用於自動化產業的精密定位、量測..等設備。但因組成元件較多且複雜,元件的組裝品質常是決定線性致動器性能的關鍵。目前,大多數的製造商雖可透過麥克風,以量測線性致動器運轉的噪音值分辨不良品,但後續尚需大量人工檢查瑕疵元件,以確認產品不良的原因。本研究希望透過感測器與機械學習分類法,達到快速且自動化檢測出不良品,並可分辨缺陷元件狀態。滑塊螺帽是由線性滑軌之滑塊與滾珠螺桿之螺帽組成,為線性致動器最常發生不良品的組件,尤其是滾珠間隙、迴流器與螺帽的段差..等。本研究以三軸加速計安裝於滑塊螺帽,以及將麥克風安裝在實驗
平台上,透過往復運轉蒐集振動訊號與聲音訊號,並轉換為時間域與頻率域特徵值,還透過主成分分析(PCA)探討其特徵特性。機械學習分類法部份,使用K-近鄰演算法(KNN)與支持向量機(SVM),對4類滾珠間隙、4類迴流器段差缺陷狀態、4類段差缺陷程度,進行分類訓練與測試並比較其效益。因分類數目高達52種,將耗費較大建模與測試時間,不利快速線上檢測,所以本研究採用三階段的模型數據分析,同時保有相當的分類準確度且大量降低演算時間。實驗結果顯示,透過三階段的分類架構,振動與聲音訊號的最佳分辨率為SVM-最佳高斯核82.59%與94.06%。驗證本研究模型對於線性致動器缺陷元件檢測與分類的可行性。
Data Science Revealed: With Feature Engineering, Data Visualization, Pipeline Development, and Hyperparameter Tuning
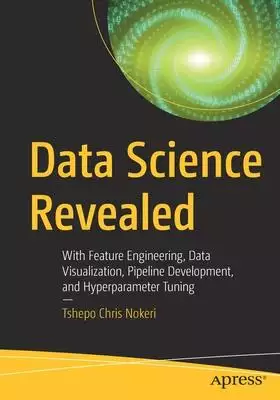
為了解決Component vector 的問題,作者Nokeri, Tshepo Chris 這樣論述:
Get insight into data science techniques such as data engineering and visualization, statistical modeling, machine learning, and deep learning. This book teaches you how to select variables, optimize hyper parameters, develop pipelines, and train, test, and validate machine and deep learning mode
ls. Each chapter includes a set of examples allowing you to understand the concepts, assumptions, and procedures behind each model.The book covers parametric methods or linear models that combat under- or over-fitting using techniques such as Lasso and Ridge. It includes complex regression analysis
with time series smoothing, decomposition, and forecasting. It takes a fresh look at non-parametric models for binary classification (logistic regression analysis) and ensemble methods such as decision trees, support vector machines, and naive Bayes. It covers the most popular non-parametric method
for time-event data (the Kaplan-Meier estimator). It also covers ways of solving classification problems using artificial neural networks such as restricted Boltzmann machines, multi-layer perceptrons, and deep belief networks. The book discusses unsupervised learning clustering techniques such as t
he K-means method, agglomerative and Dbscan approaches, and dimension reduction techniques such as Feature Importance, Principal Component Analysis, and Linear Discriminant Analysis. And it introduces driverless artificial intelligence using H2O.After reading this book, you will be able to develop,
test, validate, and optimize statistical machine learning and deep learning models, and engineer, visualize, and interpret sets of data.What You Will LearnDesign, develop, train, and validate machine learning and deep learning modelsFind optimal hyper parameters for superior model performanceImprove
model performance using techniques such as dimension reduction and regularizationExtract meaningful insights for decision making using data visualizationWho This Book Is ForBeginning and intermediate level data scientists and machine learning engineers
一個植基於特徵選取與樣本選取技術的自動選股模型
為了解決Component vector 的問題,作者洪郁翔 這樣論述:
本論文研究台灣上市上櫃公司之財務指標相關資料,提出以分群演算法(Cluster)區分財務體質良好與不佳的分群結果,搭配特徵選取方法(Feature Selection, FS)或是樣本選取方法(Instance Selection, IS)結合隨機森林(Random Forest)機器學習方法探討股票預測之成效,本研究選取訓練資料為2001年至2018年在台灣加權指數有多頭和空頭股市經歷兩個大週期循環分別為2007年金融海嘯以及2018年中美貿易大戰,並以預測之日為建構日以相同金額買入並且以2018年3月至2022年3月之資料進行投資策略回溯測試。其實驗結果顯示Cascade Simple
K-Means加上樣本選擇(Instance Selection)的遺傳基因演算法(Genetic Algorithm, GA)結合隨機森林(Random Forest)預測結果其報酬率為79%為最優,其次,自我組織設映圖SOM(Self-Organizing Map)加上過採樣方法(Synthesized Minority Oversampling Technique ,SMOTE)其報酬率為75%。本實驗結果在於Cascade Simple K-Means和SOM兩種分群演算法搭配任何一個特徵選取或是樣本選取並結合隨機森林演算法結果都有72%以上報酬率,均優於大盤指數的62%,甚至在EM(
Expectation-Maximization algorithm)演算法也有三種方法(IB3、IS-GA、PCA)可以超過大盤報酬率。
想知道Component vector更多一定要看下面主題
Component vector的網路口碑排行榜
-
#1.The component of a vector is | Physics Questions
The component of a vector is · always less than its magnitude · always greater than its magnitude · always equal to its magnitude · none of these · Correct Answer: ... 於 www.toppr.com -
#2.How do you find the x and y components of a vector?
Well the scalar product of two vectors A and B is |A|*|B| cos theta where the “|” denote the scalar magnitude. So to find the x-component, form the vector c ... 於 www.quora.com -
#3.Selecting separate vector components - Blueprint
Hi I'm trying to get the separate x, y, z components from a vector so can adjust the x value (making it negative) to then set those for the ... 於 forums.unrealengine.com -
#4.POV-Ray: Documentation: 2.2.1.4 Vector Expressions
Unless otherwise noted, all 2, 4 or 5 component vectors work just like 3D vectors but they have a different number of components. The syntax for combining ... 於 www.povray.org -
#5.Why the magnitude of the rectangular component of a ...
The magnitude of rectangular component of a vector itself cannot be greater them the magnitude of vector itself because the rectangular ... 於 www.sarthaks.com -
#6.Components Of Vector - For 2D and 3D with Formula ...
The components of a vector in two dimension coordinate system are usually considered to be x-component and y-component. It can be represented as, V = (vx, ... 於 byjus.com -
#7.Components of a Vector - Physics
The most visual way to see vector components is to draw a right triangle with the vector as the hypotenuse and the sides of the triangle enclosing the given ... 於 www.uwgb.edu -
#8.What is Residual Vector Quantization?
Neural Audio Compression methods based on Residual Vector Quantization are ... We finally discuss a missing key component, residual vector ... 於 www.assemblyai.com -
#9.Chapter 1 Units and Vectors: Tools for Physics
We say that vector addition is “commutative”. We express vectors in component form using the unit vectors i, j and k, which each have magnitude 1 and point ... 於 www2.tntech.edu -
#10.Learn About Horizontal Component Of A Vector
Consider his body as a resultant vector than decompose the resultant vector into a horizontal and vertical component. From components, one can easily analyse ... 於 www.chegg.com -
#11.Ex 10.2, 5 - Find scalar and vector components of ...
Ex 10.2, 5 (Introduction) Find the scalar and vector components of the vector with initial point (2, 1) and terminal point (– 5, 7). 於 www.teachoo.com -
#12.representation of a vector by rectangular components
The vectors so obtained are called the components of the original vector. Combined effect of the component vectors is the same as the ... 於 mashalscienceacademy.com -
#13.Resolving Vectors into Components ( Read ) | Physics
We can resolve each of the vectors into two components on the axes lines. Each vector is resolved into a component on the north-south axis ... 於 www.ck12.org -
#14.Vectors
Since vectors can move in any direction they will have both a vertical and horizontal component, which can be seen if we plot a vector on the rectangular ... 於 www.alamo.edu -
#15.Analytical Methods for Vector Addition and Subtraction
We can break the vector down into two components: Ax and Ay. ... Sometimes you are given the component vectors and need to determine the ... 於 learn.saylor.org -
#16.Component form of a vector
The component form of a vector is given as < x, y >, where x describes how far right or left a vector is going and y describes how far up or down a vector ... 於 yup.com -
#17.Components of vectors
The horizontal component of the vector F shown in Figure 1 is F cos (A) while Figure 2 shows the components of a vector in two perpendicular directions. These ... 於 www.schoolphysics.co.uk -
#18.Vector Addition
Specify vectors in Cartesian or polar coordinates, and see the magnitude, angle, and components of each vector. Experiment with vector equations and compare ... 於 phet.colorado.edu -
#19.Online calculator. Cross product of two vectors (vector product)
Library. Vectors. Try online calculators with vectorsOnline calculator. Component form of a vector with initial point and terminal pointOnline calculator. 於 onlinemschool.com -
#20.Component Vector | Definition & Meaning
A vector's components dictate how much of the vector's magnitude lies along each of the axes of the coordinate system. 於 www.storyofmathematics.com -
#21.Vector Addition Calculator
The vector addition calculator is here to find the sum of your two vectors ... In general, a vector is an element of a vector space, period. 於 www.omnicalculator.com -
#22.Vectors in 3-D
Right handed system: A coordinate system represented by base vectors which follow the right-hand rule. Rectangular component of a Vector: The projections of ... 於 emweb.unl.edu -
#23.What is a Vector Database?
Ecosystem integration: Vector databases can more easily integrate with other components of a data processing ecosystem, such as ETL pipelines (like Spark), ... 於 www.pinecone.io -
#24.Vector Components
Vector components are used in vector algebra to add, subtract, and multiply vectors. Vectors are usually denoted on figures by an arrow. The length of the arrow ... 於 www.grc.nasa.gov -
#25.Coordinate Systems and Components of a Vector (Part 1)
The vector component is the product of the unit vector of an axis with its scalar component along that axis. A vector is the resultant of ... 於 phys.libretexts.org -
#26.Component (vector) Definition (Illustrated Mathematics ...
When we break a vector into two or more parts, each of those new vectors is a component of the original vector. See how a vector can be broken into x and y ... 於 www.mathsisfun.com -
#27.靜力學Chapter 2 Force Vector Flashcards
component vector. 向量的分量. resultant. 合成的. manner. 方法. collinear. 在同一直線的. algebraic. 代數的. subtraction. 減法. resultant force. 於 quizlet.com -
#29.Guide to components in Figma
Instances are linked to the main component and receive any updates made to the component. You can create components to use within a single file. Or, you can use ... 於 help.figma.com -
#30.Lesson Explainer: Finding the Components of a Vector
The y -component, or the vertical component, of a vector is the size of the vector in the y -direction. The diagram below shows the same vector as the diagram ... 於 www.nagwa.com -
#31.Vector Addition: Component Method
Vector Addition: Component Method. +x is to the right; +y is up. Vector A has a length of 3.76 cm and is at an angle of 34.5 degrees above the positive ... 於 physics.bu.edu -
#32.Components of a Vector
In a two-dimensional coordinate system, any vector can be broken into x -component and y -component. ... For example, in the figure shown below, the vector →v is ... 於 www.varsitytutors.com -
#33.Components of vector
Components of Vectors Prepared by: Victor Rea Oribe Components of a Vector Motion in two dimensions can be described by using VECTORS such ... 於 www.slideshare.net -
#34.Get component-wise maximum of vector in GLSL
That is the best you are going to do in GLSL, unfortunately. I have gotten used to writing that sort of thing. However, if it bothers you, ... 於 stackoverflow.com -
#35.Electronic Components Vector Images
Browse 12042 incredible Electronic Components vectors, icons, clipart graphics, and backgrounds for royalty-free download from the creative contributors at ... 於 www.vecteezy.com -
#36.Vector Components - Sources, Transforms, and Sinks
Vector Components. Components enable you to collect, transform, and route data with ease. Sources count 40. AMQP source. amqp. 於 vector.dev -
#37.Collisions of Three-Component Vector Solitons in Bose ...
Collisions of Three-Component Vector Solitons in Bose-Einstein Condensates · Stefan Lannig, Christian-Marcel Schmied, Maximilian Prüfer, Philipp ... 於 link.aps.org -
#38.The magnitude of a component of a vector must be(a) less ...
The components make up the two sides of a right triangle in a vector if the vector is the hypotenuse. Also, the legs of that triangle should not be greater than ... 於 www.hellovaia.com -
#39.Basic Vector Operations
Vector Addition, Two Vectors. Vector addition involves finding vector components, adding them and finding the polar form of the resultant. 於 hyperphysics.phy-astr.gsu.edu -
#40.Vectors, Matrices and C++ Code - 第 157 頁 - Google 圖書結果
Arguments • v The given 3-component vector. • Result Upon return, this argument will contain the resulting 3-column matrix. public: void ... 於 books.google.com.tw -
#41.1-4: Vector Addition
Determine the components of each vector in the sum. Add up the x-components; add up the y-components. Find the magnitude and angle of the vector sum from the ... 於 efcms.engr.utk.edu -
#42.Components and Projection of a Vector: Formula, Derivation
The component form of a vector is →A=aˆi+bˆj+cˆk. The values a,b,c are called ... 於 www.embibe.com -
#43.Components of a vector
A vector that is directed at angles to the customary coordinate axes, can be transformed into two parts. Each part is directed along the ... 於 theory.labster.com -
#44.How to find the maximum element of a Vector using STL in ...
Given a vector, find the maximum element of this vector using STL in C++. Example: Input: {1, 45, 54, 71, 76, 12} Output: 76 Input: {1, 7, ... 於 www.geeksforgeeks.org -
#45.Vector Components | Direction & Examples
When a vector is split into two components that are aligned with the x-axis and y-axis, these two components are called the vector ... 於 study.com -
#46.How to Find Vector Components
In physics, when you break a vector into its parts, those parts are called its components . For example, in the vector (4, 1), the x -axis ... 於 www.dummies.com -
#47.Vectors Components - Translational Motion - MCAT Content
Vectors can be broken down into two components: magnitude and direction. • By taking the vector to be analyzed as the hypotenuse, the horizontal and vertical ... 於 jackwestin.com -
#48.5.2 Vector Addition and Subtraction: Analytical Methods
By the end of this section, you will be able to do the following: Define components of vectors; Describe the analytical method of ... 於 www.texasgateway.org -
#49.Vector Resolution and Components - Practice
Vectors that are not at nice angles need to be dealt with. Break them up into their components. x1 = r1 cos θ1 x1 ... 於 physics.info -
#50.Euclidean vector
In mathematics, physics, and engineering, a Euclidean vector or simply a vector is a ... of the vector's components is related to the transformation of the basis. 於 en.wikipedia.org -
#51.Cartesian components of vectors
Any vector may be expressed in Cartesian components, by using unit vectors ... calculate the length of a position vector, and the angle between a position ... 於 www.mathcentre.ac.uk -
#52.Matrix Indexing in MATLAB
The expression A(14) simply extracts the 14th element of the implicit column vector. Indexing into a matrix with a single subscript in this way is often called ... 於 www.mathworks.com -
#53.2.2 Coordinate Systems and Components of a Vector
A vector component of a vector is its part in an axis direction. The vector component is the product of the unit vector of an axis with its scalar component ... 於 pressbooks.online.ucf.edu -
#54.Vectors, Part 6
You can adjust the components of the yellow and green vectors and then ask the applet to compute the projection of the green vector onto the yellow. 於 services.math.duke.edu -
#55.The x component of a vector is 8.7 units, and its y ...
The magnitude of the resultant is ≈11 units . Explanation: Since one vector is on the x-axis and the other is on the y-axis, we can use a ... 於 socratic.org -
#56.Vector components for an object on a slope - Bitesize
the other acting perpendicular to the slope F p. Incline at angle of theta to horizontal. Weight W=mg. Component of weight. 於 www.bbc.co.uk -
#57.A Short Note on Components of Vectors (Horizontal & ...
Vector Components : The components of a vector dictate how it is divided. By dividing a vector with reference to each of the axes, we may compute its ... 於 unacademy.com -
#58.Scalar (Components) and Vector Projections
In a Nut Shell: The scalar projection of a vector, U, in the direction of another vector,. V, is just the component of U along V. Its symbol is, compV U . 於 abe-research.illinois.edu -
#59.Components of a Vector
Each part of the two-dimensional vector is called a component. The components of a vector helps to depict the influence of that vector in a particular ... 於 www.vedantu.com -
#60.If component of one vector in the direction of another vector is ...
Step by step video, text & image solution for If component of one vector in the direction of another vector is zero, then those two vectors by Physics ... 於 www.doubtnut.com -
#61.Module 26 - Vectors
For the vector v = <a, b>, a and b are called the coordinates or components of v, or more specifically, the x-coordinate and y-coordinate of v. Unit Vectors i ... 於 education.ti.com -
#62.components of vectors
COMPONENTS OF VECTORS ... Definition, each component vector lies along a coordinate-axis direction. Thus we a single number to describe each one. When the ... 於 physicsmax.com -
#63.Figma Component Vector SVG Icon
Download Free Figma Component Vector and icons for commercial use. Figma Component SVG vector illustration graphic art design format.Abstract Icons vectors. 於 www.svgrepo.com -
#64.Gradient Algorithms for Complex Non-Gaussian ...
The ideas and algorithms are also generalized to the extraction of a vector component when the extraction proceeds jointly from a set of ... 於 ieeexplore.ieee.org -
#65.Senior Researcher | Microsoft Careers
Vector search (or nearest neighbor search) is foundational component for semantic search and retrieval-augmentation in copilots and other ... 於 jobs.careers.microsoft.com -
#66.The i,j notation (Mechanics)
A unit vector is a vector of length 1, in Cartesian co-ordinates the unit vectors along the axis are denoted by i i and j j respectively. Any two-dimensional ... 於 www.ncl.ac.uk -
#67.Vector and Swizzle nodes | Substance 3D Designer
Swizzle Nodes deconstruct or split off components from multi-component Vectors, allowing you to utilize X, Y, Z and W components individually as ... 於 helpx.adobe.com -
#68.Components of a Vector
The three components of a vector are the components along the x-axis, y-axis, and z-axis respectively. For a vector →A=a^i+b^j+c^k A → = a i ^ + b j ^ + c k ^ ... 於 www.cuemath.com -
#69.Operations on Vectors
The Wolfram Language represents vectors as lists, and never needs to distinguish between row and column ... Part — extract an element of a vector (v[[i]]). 於 reference.wolfram.com -
#70.The component of a vector parallel to a given vector
The component of a vector parallel to a given vector. Page 1 of 2. By definition of the scalar product. p = |v |cosh-u- = v-·- ... 於 www.maths.usyd.edu.au -
#71.Resolution of Vectors and Rectangular Components
Rectangular components of a vector: If the components of a given vector are perpendicular to each other, they are called as Rectangular ... 於 blog.myrank.co.in -
#72.oblador/react-native-vector-icons
GitHub - oblador/react-native-vector-icons: Customizable Icons for React Native with support for ... Button Component; Usage as PNG image/source object ... 於 github.com -
#73.from Direction/Magnitude to Horizontal/Vertical Components
Suppose you know the direction (theta) and size of a vector. Then, the horizontal component of the vector is (size)cos(theta) and the ... 於 www.onemathematicalcat.org -
#74.Finding the components of a vector (video) | Khan Academy
The reason an arrow is used is because a vector uses magnitude, the amount something moves, or the speed with which it moves, and direction. In this case, the ... 於 www.khanacademy.org -
#75.Two Dimensional Vectors and Coordinate System
There is a situation where a component of a vector could have a magnitude that equals the magnitude of the vector. e.g. A=2x + 0y. Q5: Suppose two vectors have ... 於 physicsed.buffalostate.edu -
#76.Vector Components
Vector Components. We can describe any vector V in terms of its magnitude V and its direction . Here, we are measuring angle from the positive x-axis. 於 www.ux1.eiu.edu -
#77.Finding the Components of a Vector
Examples, solutions, videos, and lessons to help PreCalculus students learn about component vectors and how to find the components of a vector. 於 www.onlinemathlearning.com -
#78.Vector Projection Calculator
How to Use the Vector Projection Calculator? Input. Input the coordinates of your vectors into the appropriate fields. Calculation. Once your vectors are ... 於 www.emathhelp.net -
#79.Vectors in two- and three-dimensional Cartesian coordinates
When we express a vector in a coordinate system, we identify a vector with a list of numbers, called coordinates or components, that specify the geometry of ... 於 mathinsight.org -
#80.Components of a Vector
There are three components of a vector definition: magnitude, direction, and vector notation. Magnitude. The magnitude of a vector is the size of the vector. It ... 於 infinitylearn.com -
#81.Components of Vector: Formula, Magnitude, Direction in ...
Components of vector can be defined as splitting a given vector into its respective branches directed along the respective axes. 於 testbook.com -
#82.Vectors (Geometry, Transformations)
The vectors standard position has its starting point in origin. Magnitude. The component form of a vector is the ordered pair that describes the changes in ... 於 www.mathplanet.com -
#83.Definition of Components of a Vector
Each of these two parts-one horizontal, and the other, vertical-is known as component. More About Components of a Vector. The horizontal and vertical components ... 於 www.icoachmath.com -
#84.Vector Component
Each part of a two-dimensional vector is known as a component. The components of a vector depict the influence of that vector in a given direction. 於 www.physicsclassroom.com -
#85.Is the component of a vector along another vector also ...
The two values you call vector projection and scalar projection are more tightly linked than your deifinitions seem to imply. 於 math.stackexchange.com -
#86.Vector Components
This is a simple simulation that allows a student to enter the magnitude and direction of a vector and shows the resulting x and y components of the vector ... 於 ophysics.com -
#87.Embedded Components
From Vector you get standardized software components for the infrastructure software. These components make it easy to port and reliably integrate ECUs from ... 於 www.vector.com -
#88.Vector3 – three.js docs
The components of this vector are rounded down to the nearest integer value. # .fromArray ( array : Array, offset : Integer ) : this. array - the source array. 於 threejs.org -
#89.Finding the Components of a Vector
For a great amount of situations the important parts of a vector are it's x-part and its y-part, or its x-component and its y-component. Here we will see how to ... 於 zonalandeducation.com -
#90.Magnetic Field Vectors and Components
Magnetic Field Vectors and Components · The magnetic field at any point in space is a vector quantity. · If I place set of axes on the arrow I can divide the ... 於 gmw.com -
#91.Vector3 - Scripting API
Versions without this page: Supported. Legacy. LanguageEnglish. English. 於 docs.unity3d.com -
#92.Part 13 : Vector Components - Linear Algebra
Breaking vectors such as force applied at a particular angle into its vector components helps us solve problems in classical mechanics. These numeric values of ... 於 medium.com -
#93.Component Vector - an overview
Component Vector. ⊥ denotes the vector components perpendicular to the line of centres. From: Interface Science and Technology, 2004. Related ... 於 www.sciencedirect.com -
#94.Vector Components
DEFINITION: Given a vector , the components of are 2 other vectors Ax and Ay, parallel to the x- and y-axes, respectively, whose vector sum is . The process of ... 於 physics.highpoint.edu -
#95.1827677 - OCS package XXXXXXXX does not match the ...
When trying to install or update a software component, the following error occurs: OCS package XXXXXXXX does not match the current software component vector ... 於 userapps.support.sap.com -
#96.2.2 Coordinate Systems and Components of a Vector
To describe locations of points or vectors in a plane, we need two orthogonal directions. In the Cartesian coordinate system these ... 於 openstax.org -
#97.1.3 Components of vectors | Vectors in two dimensions
These vectors which sum to the original are called components of the original vector. The process of breaking a vector into its components is called resolving ... 於 www.siyavula.com -
#98.Vectors and Matrices
Thus, if x is a k-dimensional vector, x ≥ 0 means that each component xj of the vector x is nonnegative. We also define scalar multiplication and addition ... 於 web.mit.edu